AI is revolutionizing predictive maintenance by enabling manufacturers to forecast equipment failures before they occur. By analyzing real-time data from IoT sensors and historical records, AI helps identify potential issues early, reducing downtime and repair costs. In this blog, we’ll explore how AI technologies, such as machine learning and data analytics, empower manufacturers to optimize maintenance schedules, improve equipment lifespan, and enhance overall operational efficiency.
Table of Contents
- Overview of Predictive Maintenance
- Importance of Predictive Maintenance
- Understanding Predictive Maintenance
- The Role of AI in Predictive Maintenance
- Data Sources for Predictive Maintenance
- AI Techniques for Predicting Equipment Failures
- Benefits of Using AI for Predictive Maintenance
- Challenges in Implementing AI for Predictive Maintenance
- Case Studies: Successful Implementation of AI in Predictive Maintenance
- Future Trends in AI and Predictive Maintenance
- Enhancing Predictive Maintenance using AI, IoT, and Custom Solutions with HashStudioz
- Conclusion
Overview of Predictive Maintenance
Equipment failures in manufacturing can lead to significant disruptions, resulting in lost productivity and increased operational costs. Understanding the causes and implications of these equipment failures is crucial for manufacturers aiming to maintain operational efficiency and competitiveness.
Importance of Predictive Maintenance
Predictive maintenance (PdM) is a proactive maintenance strategy that enables manufacturers to predict equipment failures before they happen. By leveraging advanced technologies like AI, IoT sensors, and machine learning, manufacturers can significantly reduce unplanned downtime, cut maintenance costs, and extend the lifespan of critical machinery. This approach not only enhances equipment reliability but also boosts overall productivity and operational efficiency, making predictive maintenance a key driver of long-term success in manufacturing operations.
Understanding Predictive Maintenance
Definition and Key Concepts
Predictive maintenance involves the use of data analytics and machine learning to predict when equipment is likely to fail. This approach contrasts with traditional maintenance strategies, which may rely on scheduled checks or reactive repairs, making predictive maintenance a more efficient solution.
Differences Between Predictive, Preventive, and Reactive Maintenance
- Predictive Maintenance: Uses data to forecast failures and schedule maintenance accordingly, optimizing resource allocation.
- Preventive Maintenance: Involves regular maintenance tasks based on time intervals or usage metrics, which may not always align with actual equipment needs.
- Reactive Maintenance: Focuses on repairing equipment after a failure has occurred, often leading to costly downtime.
The Role of AI in Predictive Maintenance
How AI Algorithms Analyze Data
AI algorithms play a crucial role in predictive maintenance by processing vast amounts of data from various sources. They identify patterns that indicate potential equipment failures, enabling manufacturers to make informed decisions about maintenance schedules.
Machine Learning and Its Applications in Maintenance
Machine learning models can learn from historical data, improving their predictive accuracy over time. These models adapt to new data, ensuring that predictions remain relevant as equipment and operating conditions change, thus enhancing the effectiveness of predictive maintenance strategies.
Data Sources for Predictive Maintenance
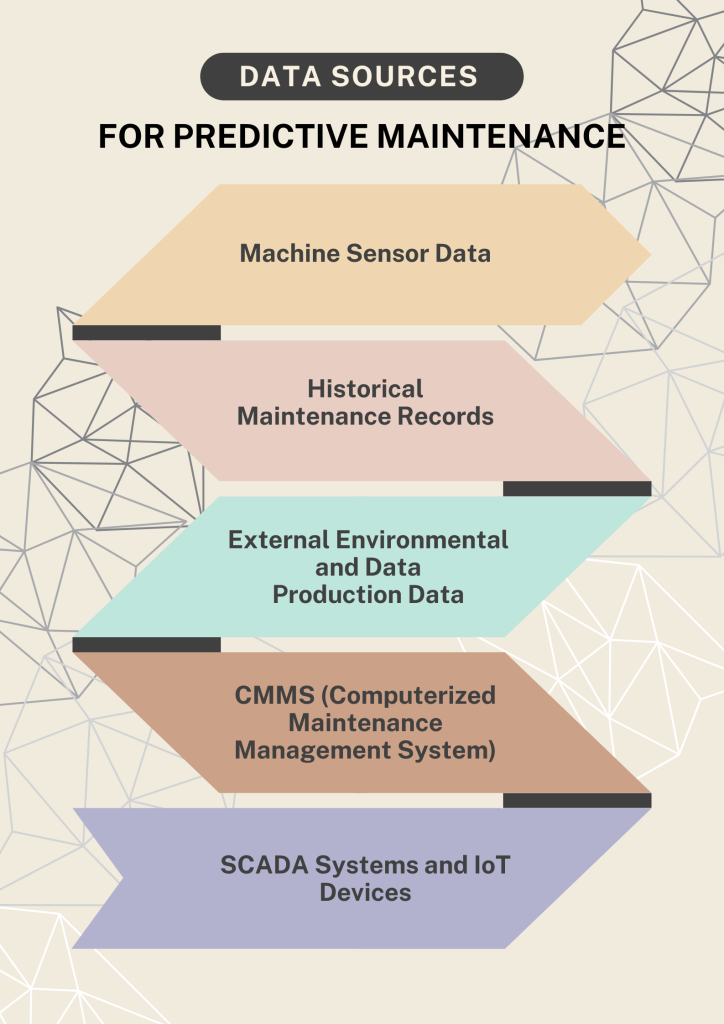
1. Sensors and IoT Devices in Predictive Maintenance
IoT devices and sensors are essential for collecting real-time data on equipment performance, including critical metrics such as temperature, vibration, and pressure. This data is crucial for effective predictive maintenance. By integrating data analytics services, businesses can process and analyze real-time information to implement proactive maintenance strategies. This approach reduces unplanned downtime, extends equipment lifespan, and optimizes operational efficiency, leading to improved productivity and cost savings.
2. Historical Maintenance Records for Predictive Maintenance
Analyzing historical maintenance records allows manufacturers to identify trends and patterns that are key to building accurate predictive models. By understanding how equipment has behaved over time, businesses can enhance the precision of their predictive maintenance efforts, helping to schedule timely interventions and prevent unexpected failures.
3. Environmental and Operational Data for Accurate Predictive Models
External factors like environmental conditions and operational parameters significantly affect equipment performance. Incorporating this environmental and operational data into predictive models improves their accuracy and reliability, making predictive maintenance even more effective and enabling manufacturers to better plan for maintenance needs, reduce costs, and boost efficiency.
AI Techniques for Predicting Equipment Failures
1. Anomaly Detection
Anomaly detection algorithms identify deviations from normal operating conditions, signaling potential issues before they escalate into equipment failures. This proactive approach is a cornerstone of effective predictive maintenance.
2. Time Series Analysis
Time series analysis examines data points collected over time to identify trends and patterns that can predict future equipment behavior. This technique is crucial for developing accurate predictive maintenance strategies.
3. Predictive Modeling
Predictive modeling uses statistical techniques and machine learning to forecast when equipment is likely to fail, allowing for timely maintenance interventions and reducing the risk of unplanned downtime.
Benefits of Using AI for Predictive Maintenance
1. Reduced Downtime and Increased Efficiency
By predicting equipment failures, manufacturers can schedule maintenance during non-peak hours, minimizing disruptions to production and enhancing overall operational efficiency.
2. Cost Savings and Resource Optimization
Focusing maintenance efforts on actual equipment needs reduces unnecessary costs associated with over-maintenance and emergency repairs, leading to significant cost savings.
3. Enhanced Safety and Compliance
Predictive maintenance improves workplace safety by identifying potential hazards before they lead to accidents, ensuring compliance with safety regulations and fostering a safer work environment.
Challenges in Implementing AI for Predictive Maintenance
1. Data Quality and Integration Issues
For accurate predictions in AI in predictive maintenance, high-quality data is essential. Manufacturers may encounter challenges when integrating AI solutions with existing systems and ensuring consistent data flow. Without reliable data, predictive models may lose accuracy, hindering the effectiveness of predictive maintenance strategies.
2. Overcoming Resistance to Change in Organizational Culture
Adopting AI in predictive maintenance may face resistance from employees who are accustomed to traditional maintenance practices. Successful implementation requires effective change management strategies to foster acceptance and ensure smooth adoption of AI-driven solutions across the organization.
3. Bridging the Skills Gap for AI in Predictive Maintenance
A significant barrier to AI in predictive maintenance is the lack of skilled personnel to manage and interpret AI systems. Investing in comprehensive training and development programs is essential for building a knowledgeable workforce capable of maximizing the potential of AI technologies in predictive maintenance.
Case Studies: Successful Implementation of AI in Predictive Maintenance
Example 1: Manufacturing Industry
A leading automotive manufacturer implemented AI-driven predictive maintenance, resulting in a 30% reduction in unplanned downtime and significant cost savings, showcasing the effectiveness of predictive maintenance strategies.
Example 2: Energy Sector
An energy company utilized predictive maintenance to monitor turbine performance, leading to improved reliability and a decrease in maintenance costs, demonstrating the value of AI in predictive maintenance.
Example 3: Transportation and Logistics
A logistics provider adopted AI for predictive maintenance on its fleet, enhancing vehicle uptime and reducing operational costs, further illustrating the benefits of predictive maintenance.
Future Trends in AI and Predictive Maintenance
1. Advancements in AI Technologies
Emerging AI technologies, including deep learning and advanced analytics, are set to significantly enhance predictive maintenance capabilities. These innovations will improve the accuracy and efficiency of maintenance predictions, enabling businesses to minimize downtime and reduce operational costs. By leveraging AI development services, companies can harness the power of these advanced technologies to optimize asset management, automate processes, and drive more reliable, data-driven decision-making.
2. The Role of Big Data and Cloud Computing
The integration of big data and cloud computing will facilitate the storage and analysis of vast datasets, improving predictive accuracy and enabling real-time collaboration among teams, which is essential for effective predictive maintenance.
3. Integration with Industry 4.0
As manufacturers embrace Industry 4.0, the convergence of AI, IoT, and automation will drive further advancements in predictive maintenance strategies, allowing for smarter and more connected manufacturing processes.
Enhancing Predictive Maintenance using AI, IoT, and Custom Solutions with HashStudioz
1. AI & Machine Learning
HashStudioz leverages machine learning algorithms to analyze large volumes of data from equipment sensors and historical maintenance records. Predictive models forecast potential equipment failures, allowing manufacturers to take proactive action and schedule maintenance before issues escalate. This data-driven approach helps optimize asset utilization and minimize downtime.
2. IoT Integration
HashStudioz offers IoT-based solutions for real-time equipment monitoring. By integrating IoT devices, manufacturers can track key parameters like temperature, vibration, and pressure, providing valuable insights into equipment health. Additionally, seamless connectivity ensures smooth data flow between IoT devices and central systems, enhancing the accuracy of predictive analytics.
3. Custom Software Solutions
HashStudioz develops tailored software solutions that align with specific business needs. These applications integrate predictive maintenance features, enabling manufacturers to monitor equipment status effectively. User-friendly dashboards and reporting tools are designed to simplify data interpretation, helping maintenance teams make informed decisions based on real-time insights.
4. Analytics & Reporting
Advanced analytics tools offered by HashStudioz help visualize equipment data trends and identify patterns that indicate potential failures. Automated reporting systems reduce the manual effort required by maintenance teams, delivering actionable insights based on real-time data. These tools improve efficiency by streamlining decision-making and ensuring timely intervention.
5. Continuous Support
HashStudioz ensures continuous improvement of predictive models by retraining them with new data and feedback, maintaining high accuracy levels. Along with ongoing support, we offer training programs for staff to ensure they are equipped to use the latest technologies and leverage predictive maintenance tools effectively, leading to long-term operational success.
6. Cloud Computing
By leveraging cloud computing services, HashStudioz enables scalable data storage and processing, allowing manufacturers to manage large datasets effectively. Cloud platforms also facilitate seamless collaboration among departments, ensuring that all stakeholders can access necessary insights in real-time, further improving predictive maintenance and decision-making.
This comprehensive approach empowers manufacturers to reduce unplanned downtime, extend the lifespan of equipment, and optimize maintenance schedules, leading to improved operational efficiency and cost savings.
Conclusion
Recap of the Importance of Predictive Maintenance
Predictive maintenance is a vital strategy for manufacturers seeking to optimize operations and reduce costs. By leveraging AI technologies, manufacturers can enhance their ability to predict equipment failures and implement timely maintenance interventions, ultimately leading to improved operational efficiency.
Encouragement to Embrace AI for Enhanced Operational Efficiency
Manufacturers are encouraged to adopt AI-driven predictive maintenance solutions to stay competitive in an increasingly complex market. Embracing these technologies not only improves operational efficiency but also fosters a culture of innovation and continuous improvement, positioning manufacturers for future success.