In today’s rapidly evolving technological landscape, data analytics has become a cornerstone for informed decision-making across various industries. The advent of Artificial Intelligence (AI) has further transformed this field, introducing tools and platforms that streamline data processing and analysis. Among these innovations are No-Code and Pro-Code solutions, each offering distinct approaches to data analytics. This article delves into the intricacies of these methodologies, examining their implications for data analysts and the future of data analytics services.
- 75% of enterprises are expected to shift to AI-driven data analytics by 2027.
- 65% of data analysts believe their roles will evolve rather than become obsolete due to AI.
- 80% of businesses report improved efficiency by integrating AI into their data analytics processes..
Table of Contents
- Understanding No-Code and Pro-Code Approaches
- The Rise of AI-Powered Analytics
- Comparative Analysis: No-Code vs. Pro-Code in Data Analytics
- Impact on Data Analysts in the Era of AI
- The Need for AI Model Governance
- Increased Demand for Cross-Functional Collaboration
- Future Outlook for Data Analytics Services
- Conclusion
- FAQs
Understanding No-Code and Pro-Code Approaches
Data analytics has evolved significantly, offering businesses multiple approaches to leverage data for insights and decision-making. Broadly, these methodologies can be categorized into No-Code and Pro-Code approaches. Each approach comes with its own advantages and limitations, influencing how organizations implement Data Analytics Services.
1. No-Code Platforms
No-Code platforms empower users to develop applications and perform data analytics without writing traditional code. These platforms utilize intuitive interfaces, drag-and-drop functionalities, and pre-built templates, making them accessible to individuals without programming expertise.
Key Benefits of No-Code Platforms
1. Accessibility
- Enables non-technical users, such as business analysts and marketing teams, to engage in data analytics.
- Reduces dependence on IT teams and data scientists for routine analytics tasks.
2. Rapid Deployment
- Facilitates quick development and implementation of analytics solutions.
- Allows users to create dashboards, reports, and insights within minutes.
3. Cost-Effectiveness
- Reduces the need for specialized development resources.
- Offers affordable pricing models, making it suitable for startups and SMEs.
Challenges of No-Code Platforms
Despite their advantages, No-Code platforms have limitations:
1. Limited Customization
- Constraints in tailoring solutions to specific business needs.
- Lack of flexibility in modifying algorithms and analytical models.
2. Scalability Concerns
- May struggle to handle large-scale data processing.
- Performance issues arise as organizations grow and require more advanced analytics.
3. Integration Limitations
- Potential difficulties in integrating with existing systems and workflows.
- Compatibility issues with legacy databases and third-party applications.
2. Pro-Code Solutions
Pro-Code solutions involve traditional coding practices, allowing for comprehensive customization and control over data analytics processes. These solutions are typically managed by skilled developers, data engineers, and data scientists, ensuring greater precision in analytics execution.
Key Benefits of Pro-Code Solutions
1. Deep Customization
- Enables developers to build tailored analytics solutions that meet specific business requirements.
- Allows for custom algorithms, AI models, and automation processes.
2. Scalability
- Designed to handle large-scale and complex data sets.
- Supports enterprise-level analytics with high efficiency and accuracy.
3. Integration Flexibility
- Provides seamless integration with existing systems, APIs, and cloud platforms.
- Supports multiple programming languages (Python, R, SQL) and frameworks.
Challenges of Pro-Code Solutions
1. Higher Costs
- Requires investment in skilled personnel and software infrastructure.
- Long-term costs associated with maintaining and updating solutions.
2. Longer Development Cycles
- Implementation requires thorough planning, testing, and deployment.
- Delays in rolling out new analytics features compared to No-Code platforms.
3. Maintenance Complexity
- Continuous need for system updates and performance monitoring.
- Potential challenges in troubleshooting and debugging custom-built solutions.
Data Governance and Compliance: The Role of Data Analytics Consulting Services
The Rise of AI-Powered Analytics
Artificial Intelligence (AI) has transformed Data Analytics Services, introducing cutting-edge capabilities that significantly enhance data processing, analysis, and interpretation. AI-driven analytics enables businesses to extract valuable insights from vast datasets with speed, accuracy, and automation, reducing reliance on manual efforts while improving decision-making.
1. AI in Data Analytics
AI in data analytics leverages machine learning, deep learning, and natural language processing (NLP) to enable intelligent data processing. These technologies allow systems to simulate human intelligence, identify hidden patterns, and make accurate predictions with minimal human intervention.
Key AI Technologies in Data Analytics
1. Machine Learning (ML)
- Algorithms learn from historical data and improve over time.
- Used for classification, regression, clustering, and recommendation systems.
2. Deep Learning
- Advanced ML that mimics the human brain using neural networks.
- Applied in image recognition, speech processing, and autonomous decision-making.
3. Natural Language Processing (NLP)
- Enables machines to interpret, analyze, and generate human language.
- Used in chatbots, sentiment analysis, and document summarization.
4. Computer Vision
- AI’s ability to process and analyze visual data.
- Utilized in facial recognition, medical imaging, and object detection.
5. AI-Driven Automation
- Automates repetitive analytics tasks such as data cleaning, transformation, and visualization.
- Reduces errors and speeds up decision-making.
2. Applications of AI in Data Analytics
The integration of AI in Data Analytics Services has revolutionized various business processes. Below are some of the most impactful applications:
1. Predictive Analytics
- AI models analyze historical data to forecast future trends and behaviors.
- Used in sales forecasting, demand planning, and customer behavior analysis.
2. Prescriptive Analytics
- Recommends optimal actions based on predictive insights.
- Applied in supply chain management, personalized marketing, and resource allocation.
3. Anomaly Detection
- Identifies unusual patterns and outliers in datasets.
- Crucial for fraud detection, cybersecurity, and quality control in manufacturing.
4. Natural Language Processing (NLP)
- Interprets and generates human language for better data interaction.
- Used in customer sentiment analysis, automated report generation, and AI chatbots.
5. Computer Vision
- Enables AI to process and interpret images and videos.
- Applied in self-driving cars, medical imaging, and product recognition in retail.
6. Fraud Detection
- AI analyzes transaction patterns to detect suspicious activities.
- Used in banking, insurance, and e-commerce fraud prevention.
Comparative Analysis: No-Code vs. Pro-Code in Data Analytics
A thorough comparison of No-Code and Pro-Code approaches reveals their respective strengths and limitations across various dimensions.
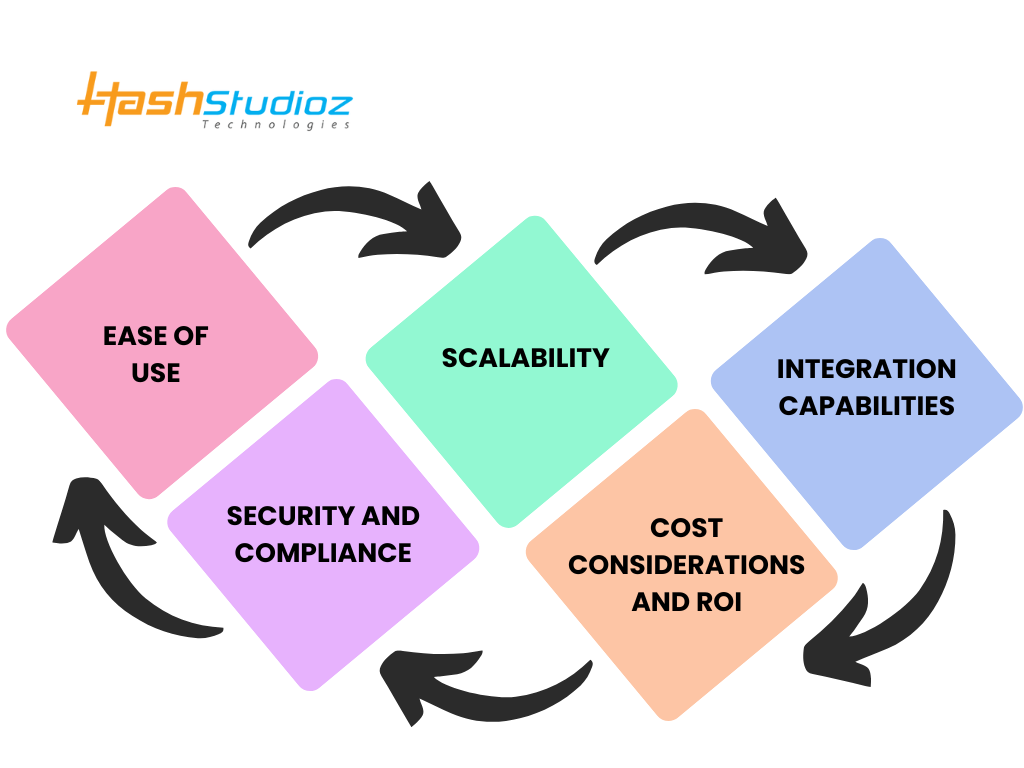
1. Ease of Use
No-Code
- User-friendly, designed for business professionals and analysts without coding expertise.
- Uses drag-and-drop interfaces, pre-built templates, and visual workflows.
- Enables quick adoption by non-technical users for tasks like reporting and dashboard creation.
Pro-Code
- Requires programming skills (Python, R, SQL) for full functionality.
- Offers more control but has a steeper learning curve.
- Preferred by data scientists, engineers, and developers for building complex models and custom analytics solutions.
2. Scalability
No-Code
- Suitable for small to medium-sized projects.
- Struggles with big data processing and complex automation.
- Limited capabilities in handling high-volume, high-velocity data.
Pro-Code
- Highly scalable, making it ideal for enterprises with vast data analytics operations.
- Can handle large datasets, machine learning models, and advanced AI-driven analytics.
- Supports cloud-based and on-premises solutions.
3. Integration Capabilities
No-Code
- Limited compatibility with legacy systems and third-party applications.
- Dependent on built-in connectors, which may restrict flexibility.
- Can face data silos due to integration challenges.
Pro-Code
- Seamless integration with databases, APIs, and enterprise solutions.
- Allows customization of data pipelines, automation workflows, and cloud-based integrations.
- Can connect with on-premise and hybrid cloud environments.
4. Security and Compliance
No-Code
- Relies on built-in security features provided by platform vendors.
- May lack advanced security controls, encryption, and data governance options.
- Compliance depends on the vendor’s adherence to industry regulations (GDPR, HIPAA, etc.).
Pro-Code
- Enables custom security measures, such as role-based access control (RBAC) and encryption.
- Ensures greater compliance with industry standards.
- More control over data privacy, authentication, and security protocols.
5. Cost Considerations and ROI
No-Code
- Lower initial costs, as it eliminates the need for hiring developers.
- Higher long-term costs due to limitations in customization and scalability.
- Prone to vendor lock-in, which can increase expenses over time.
Pro-Code
- Higher upfront investment in development resources and infrastructure.
- Better long-term ROI due to flexibility, scalability, and reduced reliance on third-party tools.
- Reduces long-term operational costs by enabling in-house customization.
Impact on Data Analysts in the Era of AI
The emergence of AI-powered analytics is reshaping the role of data analysts, rather than replacing them. While AI automates several repetitive tasks, it also presents new opportunities for analysts to focus on higher-value responsibilities, such as AI model governance, strategic decision-making, and cross-functional collaboration.
1. Automation of Routine Tasks
One of the biggest advantages of AI in Data Analytics Services is the automation of time-consuming, repetitive tasks that traditionally consumed much of a data analyst’s time.
Tasks Automated by AI
- Data Cleaning & Preprocessing: AI detects errors, removes duplicates, and normalizes data.
- Report Generation: AI-driven dashboards and reports minimize manual work.
- Anomaly Detection: AI identifies outliers and unusual patterns in real time.
- Data Transformation: AI facilitates seamless ETL (Extract, Transform, Load) processes.
Impact on Data Analysts
- Less manual effort, allowing analysts to focus on data interpretation.
- Faster processing, improving productivity and efficiency.
- More time for advanced analytics, as AI handles repetitive tasks.
2. Evolving Skill Sets
As AI-powered analytics automates basic data processing, data analysts must adapt to new skill requirements that focus on AI model management, ethical AI, and strategic decision-making.
Key Areas of Skill Evolution
1. Strategic Decision-Making
- Analysts will focus on interpreting AI-driven insights rather than manual data crunching.
- They must align AI findings with business strategies.
2. AI Model Interpretation
- Analysts must understand how AI models generate insights to ensure accuracy.
- They play a role in validating machine learning models for business use.
3. Ethical Considerations in AI
- Analysts will ensure AI models do not reinforce biases or ethical concerns.
- Data privacy and governance will become crucial responsibilities.
4. Advanced Data Storytelling
- Analysts must translate complex AI insights into actionable business recommendations.
Impact on Data Analysts
- Upskilling in AI-driven tools is essential.
- Greater focus on interpretation, validation, and ethics in AI.
- Soft skills like communication and storytelling will be critical.
3. Collaboration with AI
Rather than replacing data analysts, AI acts as an augmentation tool that enhances their productivity, accuracy, and analytical capabilities.
How Data Analysts Can Leverage AI
- Handling Large Datasets: AI speeds up data processing, allowing analysts to focus on deeper insights.
- Augmenting Decision-Making: AI suggests potential insights, which analysts validate before execution.
- Enhancing Predictive Analytics: Machine learning models predict trends, but analysts interpret and apply them.
- Customizing AI Models: Analysts adjust AI tools to align with business-specific requirements.
Impact on Data Analysts
- AI allows analysts to work smarter, not harder.
- Analysts will act as AI supervisors, ensuring models function optimally.
- The future of analytics is human-AI collaboration, not human replacement.
10 Ways to Leverage Data Analytics for Better Business Operations
The Need for AI Model Governance
As AI becomes a core component of Data Analytics Services, analysts must take responsibility for AI model governance to ensure transparency, fairness, and accountability.
Why AI Model Governance Matters
- AI Bias & Ethical Risks: AI models can reinforce biased decision-making if not properly trained.
- Regulatory Compliance: Analysts must ensure AI-driven analytics meet data privacy laws (e.g., GDPR, CCPA).
- Model Explainability: AI-generated insights must be interpretable and justifiable for business leaders.
- Continuous Model Audits: Analysts must regularly audit and retrain AI models to maintain accuracy.
Impact on Data Analysts
- Analysts must oversee AI-driven decisions to prevent biases.
- AI governance will become a key skill for modern data professionals.
- Organizations will need human oversight to maintain ethical AI standards.
Increased Demand for Cross-Functional Collaboration
AI-powered analytics is not limited to data teams—it impacts marketing, finance, operations, and product development. Data analysts will play a pivotal role in cross-functional collaboration, ensuring that AI-driven insights align with business goals.
How Data Analysts Will Work Cross-Functionally
- Marketing Teams: Providing AI-driven customer segmentation and predictive analytics.
- Finance & Risk Teams: AI-powered fraud detection and financial modeling.
- Operations Teams: AI-driven supply chain optimization and performance tracking.
- Product Development: Leveraging AI insights for user behavior analytics and feature development.
Impact on Data Analysts
- Analysts will collaborate across departments, ensuring AI insights are actionable.
- Strong business acumen will become essential.
- Data analysts will act as strategic advisors, bridging AI and business decision-making.
Future Outlook for Data Analytics Services
The landscape of data analytics services is evolving rapidly with the rise of AI-powered automation, real-time processing, and self-service analytics. However, human expertise will remain crucial in ensuring accuracy, compliance, and strategic alignment.
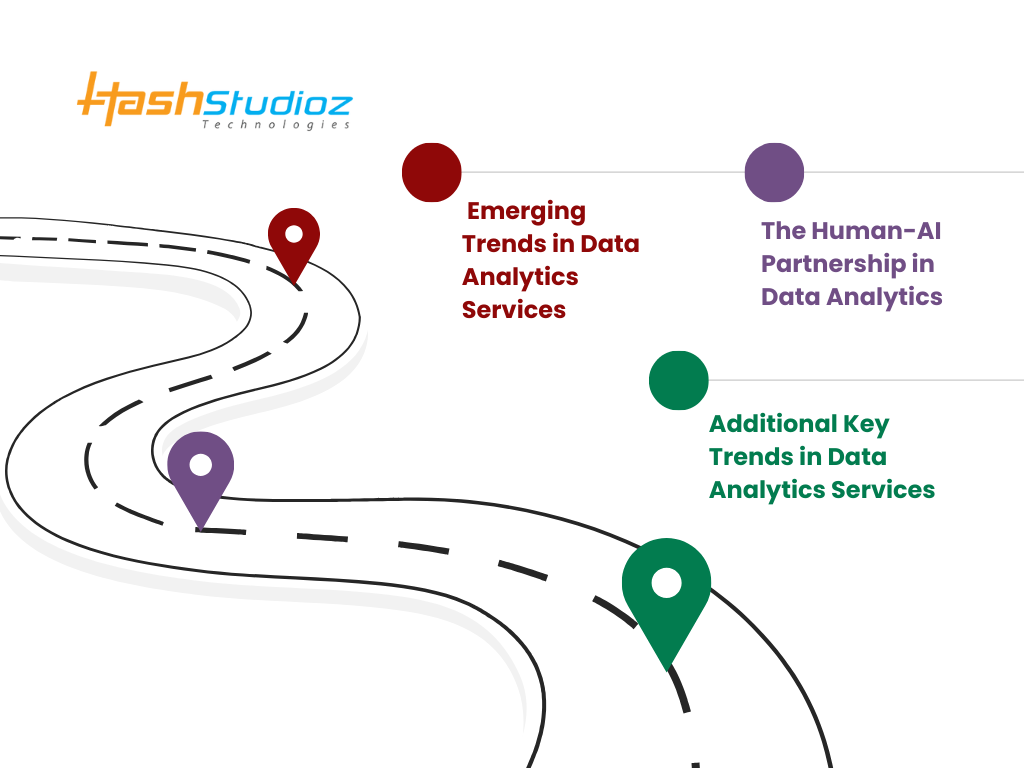
1. Emerging Trends in Data Analytics Services
AI-Driven Self-Service Analytics
- AI-powered no-code tools allow business users to generate reports and insights without technical expertise.
- This reduces dependence on data analysts for routine tasks.
- Tools like Google Looker Studio, Power BI, and Tableau are making analytics more accessible.
Explainable AI (XAI) for Trust and Transparency
- AI models must be interpretable and explainable to avoid black-box decision-making.
- Compliance with data regulations (GDPR, CCPA) is increasing demand for transparent AI models.
- XAI techniques help businesses understand why AI makes certain recommendations.
Real-Time Analytics for Instant Insights
- AI-powered streaming analytics (Kafka, Apache Spark) enable real-time data analysis.
- Businesses benefit from instant fraud detection, supply chain optimization, and customer engagement insights.
- Industries like finance, healthcare, and e-commerce rely on real-time decision-making.
2. The Human-AI Partnership in Data Analytics
Contextual Understanding & Decision-Making
- AI detects patterns, but human expertise is needed to interpret them.
- Analysts apply business knowledge to validate AI-generated recommendations.
- AI might misinterpret market anomalies or outliers, requiring human review.
Strategic Oversight in AI Analytics
- AI models optimize operations, but humans define key business objectives.
- Data analysts prioritize insights based on company strategy.
- AI lacks nuanced decision-making abilities, such as adapting to market shifts or customer sentiment.
Quality Control & Ethical Considerations
- AI models can develop biased insights if trained on skewed data.
- Data analysts ensure fair, unbiased, and compliant AI-driven analytics.
- AI must align with ethical standards, including privacy, fairness, and security.
3. Additional Key Trends in Data Analytics Services
Hybrid AI-Human Workflows
- AI automates repetitive tasks, while humans focus on strategy and insights.
- Organizations adopt AI-human collaboration models to improve efficiency.
- Data analysts fine-tune AI models and interpret insights for business impact.
Cloud-Based Data Analytics Services
- Cloud analytics platforms (AWS, Azure, Google Cloud) enable scalable, on-demand analytics.
- Big data processing is shifting to serverless cloud solutions.
- AI models are integrated into cloud platforms for enhanced data-driven decision-making.
Personalized AI-Driven Insights
- AI-powered analytics tools can deliver hyper-personalized insights based on user behavior.
- E-commerce, marketing, and finance industries are leveraging AI for customized customer experiences.
- Data analytics services will focus on predictive personalization for better engagement.
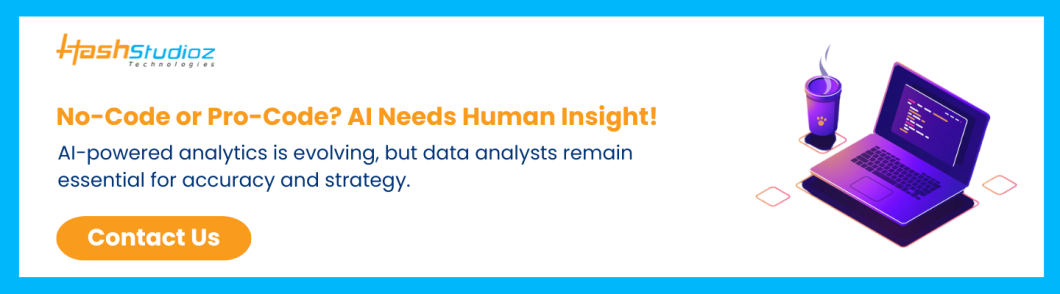
Conclusion
The debate between No-Code and Pro-Code solutions in data analytics is not about one replacing the other but rather about finding the right balance. No-Code platforms make data analytics more accessible, while Pro-Code solutions offer deep customization and scalability. AI-powered analytics enhances efficiency but does not eliminate the need for skilled data analysts. Instead, the role of data analysts is evolving, requiring them to develop advanced analytical skills and collaborate effectively with AI-driven tools. Organizations investing in Data Analytics Services must consider their specific needs to determine the optimal approach.
FAQs
1. Will AI replace data analysts?
No, AI will augment data analysts’ roles by automating routine tasks while analysts focus on strategic decision-making and complex problem-solving.
2. Are No-Code platforms suitable for enterprise-level analytics?
While No-Code platforms provide accessibility, they may lack the scalability and integration capabilities required for large enterprises.
3. What skills will data analysts need in the future?
Data analysts will need expertise in AI model interpretation, ethical AI considerations, and strategic data analysis.
4. How do No-Code and Pro-Code compare in terms of security?
Pro-Code solutions offer more advanced security controls, while No-Code platforms rely on built-in security features, which may be limited.