In an era where data is the lifeblood of decision-making, organizations are under immense pressure to keep up with evolving technologies and data infrastructures. The massive explosion of data, coupled with the need for real-time insights and cloud-native applications, has prompted businesses to rethink how they handle and leverage data. Two crucial processes—data migration and data modernization—play a central role in this evolution.
Although often misunderstood or used synonymously, these terms refer to vastly different strategies. Data migration is a tactical move involving the transfer of data from one system to another, while data modernization is a strategic initiative aimed at transforming the way an organization uses data to derive value.
To support these processes, many businesses turn to Data Analytics Consulting Services, which offer expert guidance in executing seamless data transitions and establishing modern data ecosystems tailored to business needs.
- 83% of enterprise workloads are expected to be in the cloud by 2025.
- 70% of data migration projects experience delays due to poor planning.
- Companies that modernize data infrastructure see 2.5x faster decision-making.
- Organizations using Data Analytics Consulting Services report a 3x increase in data maturity.
Table of Contents
Understanding Data Migration
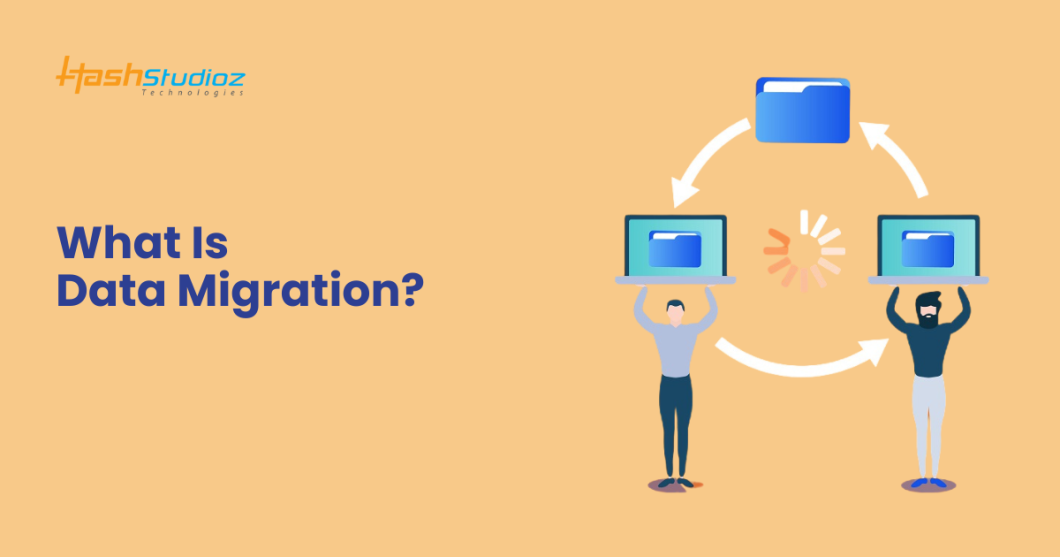
Data migration is the process of transferring data between different storage types, formats, or computing environments. Typically, this process is executed as a one-time event, often during significant IT changes such as system upgrades, cloud adoption, data center relocation, mergers, or when switching from one enterprise application to another.
At its core, data migration ensures that critical business data remains accessible, usable, and secure in the new system or environment. It involves not only moving data but also validating and transforming it to fit into the new structure.
Common Data Migration Scenarios
Organizations undertake data migration for various reasons, and the nature of the project can differ based on the use case. Some common scenarios include:
- Moving from On-Premises Systems to the Cloud: Transitioning data from traditional on-site servers to cloud environments like AWS, Azure, or Google Cloud to benefit from scalability, flexibility, and reduced maintenance overhead.
- Upgrading from Legacy Databases to Modern Platforms: Replacing outdated, monolithic databases with modern, cloud-native or distributed databases to enhance performance, support real-time analytics, and increase reliability.
- Consolidating Data Following Mergers and Acquisitions: Combining disparate data sources and systems from multiple companies into a unified, centralized infrastructure for seamless operations and unified reporting.
- Switching Enterprise Applications (e.g., ERP, CRM): Migrating data to new enterprise software to improve process automation, customer experience, and business intelligence capabilities.
Objectives of Data Migration
The main goals of a successful data migration strategy are:
- Ensuring Data Accessibility: Data should be fully available and usable in the new system with no loss in continuity.
- Minimizing Operational Disruption: Business operations must continue smoothly during and after migration, with minimal downtime or performance degradation.
- Preserving Data Integrity, Security, and Accuracy: All data must be securely transferred with strict validations to avoid corruption, duplication, or unauthorized exposure.
Key Steps in Data Migration
A structured, phased approach ensures a successful migration with minimal risk. Below are the major steps involved:
1. Planning and Assessment
- Evaluate Existing Architecture: Understand the current data landscape, including systems, formats, relationships, and volumes.
- Identify Data Sources and Dependencies: Document where all data resides and how systems interconnect, ensuring that no data is overlooked.
- Define Project Scope and Timeline: Set clear goals, constraints, and timelines while aligning with business needs.
2. Data Profiling and Cleansing
- Clean Redundant or Corrupted Data: Remove obsolete or duplicate records and correct inaccuracies to prevent transferring bad data.
- Ensure Format Consistency: Standardize units, naming conventions, and formats across data sources to streamline integration.
3. Data Mapping and Transformation
- Map Existing Structures to New Schemas: Define how each data element in the source maps to its counterpart in the destination system.
- Apply Transformation Rules: Reformat, aggregate, or split data as needed to match the new system’s requirements.
4. Testing and Validation
- Verify Data Completeness and Quality: Check whether all records were transferred correctly and without corruption.
- Validate Business Logic: Ensure that migrated data supports application functionality, reports, and business processes.
5. Execution and Cutover
- Perform the Actual Data Transfer: Execute the migration using automated tools or scripts in a controlled environment.
- Monitor for Anomalies or Errors: Watch for system failures, missing records, or format mismatches and fix them in real-time.
6. Post-Migration Audit and Optimization
- Audit for Accuracy and Compliance: Cross-check migrated data with source data and regulatory requirements.
- Fine-Tune System Performance: Optimize indexing, queries, and configurations in the new system to improve performance.
Benefits of Data Migration
When executed properly, data migration offers a range of operational and strategic benefits:
- Access to Advanced Infrastructure: Leveraging cloud platforms and modern storage systems can significantly enhance data access, processing speed, and flexibility.
- Improved System Performance: Modern environments reduce latency, increase uptime, and support high volumes of data transactions.
- Scalability and Integration: New platforms support seamless integration with third-party tools, APIs, and real-time data pipelines.
- Data Quality and Organization: The migration process often includes data cleansing, which improves overall data reliability and readiness for analytics.
Challenges of Data Migration
Despite its advantages, data migration presents several challenges that require careful planning and expert support:
- Risk of Data Loss or Corruption: Poor handling during the migration process can result in missing, misformatted, or inaccessible data.
- Downtime and Service Disruption: Improper cutover strategies can lead to business interruptions and revenue loss.
- Compatibility Issues: Source and destination systems may have incompatible data models, requiring complex transformations.
- Security and Compliance Risks: Sensitive data must be handled with utmost care to avoid breaches or violations of data protection regulations like GDPR or HIPAA.
Understanding Data Modernization
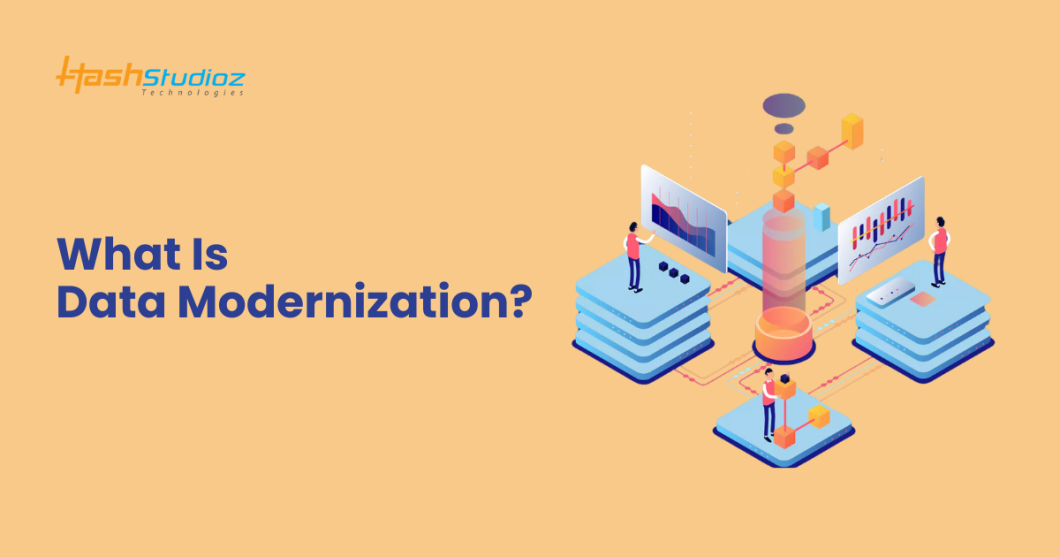
Data modernization is not merely about transferring data to new systems—it’s a transformative approach that redefines how an organization handles its data throughout its lifecycle. It involves replacing outdated, rigid, and siloed data infrastructure with modern, scalable, and cloud-native systems that are capable of supporting real-time analytics, artificial intelligence, and future-ready business strategies.
This transformation encompasses tools, technologies, methodologies, and cultural shifts aimed at enabling faster decision-making, enhanced innovation, and improved operational efficiency. Unlike data migration, which is typically a one-time event, data modernization is a continuous evolution of the enterprise’s data ecosystem.
Key Technologies in Data Modernization
Modernization initiatives are enabled by a suite of next-generation technologies that allow organizations to harness data more effectively. Some of the pivotal technologies include:
- Cloud Data Warehouses: Platforms like Snowflake, Amazon Redshift, and Google BigQuery offer elastic scaling, low-latency querying, and serverless management.
- Data Lakes and Lakehouses: Solutions like Amazon S3, Databricks Lakehouse, and Azure Data Lake combine raw storage with structured querying to support various data formats and analytics.
- Artificial Intelligence and Machine Learning (AI/ML): Integrating machine learning models enables predictive analytics, anomaly detection, and intelligent automation of decision-making processes.
- Real-time Analytics Platforms: Tools like Apache Kafka, Apache Flink, and Spark Streaming allow ingestion and processing of data streams in real time for immediate insights.
- Microservices and APIs: These facilitate modular architecture and seamless integration across diverse data sources and applications, promoting flexibility and interoperability.
Objectives of Data Modernization
A data modernization initiative is guided by a strategic vision that transcends mere technical upgrades. Its objectives include:
- Enabling Data-Driven Decision-Making: Create a robust foundation that allows leadership to make decisions backed by accurate, timely, and contextual data.
- Fostering Innovation Through Real-Time Insights: Support experimentation and innovation by enabling access to live operational data and advanced analytical models.
- Reducing Operational Costs and Increasing Agility: Leverage scalable cloud infrastructures to minimize hardware dependency, maintenance costs, and manual effort.
- Aligning Data Infrastructure with Long-Term Business Goals: Build a future-ready, flexible data architecture that can grow with evolving organizational priorities.
Key Components of Data Modernization
A successful modernization strategy comprises multiple interconnected components that work together to establish a responsive, intelligent data ecosystem.
1. Infrastructure Modernization
- Migration from Legacy Systems: Shift away from mainframes and on-premises hardware toward cloud-native infrastructure like AWS, Azure, or GCP.
- Scalable Storage and Computing: Adopt solutions that automatically scale resources up or down based on workload demand, eliminating capacity planning bottlenecks.
2. Data Architecture Redesign
- Distributed and Modular Architecture: Break monolithic data structures into modular, reusable components using microservices, containers, and orchestration platforms.
- Data Lakes and Lakehouses: Create centralized repositories for storing both structured and unstructured data, enabling unified access and analytics.
3. Data Governance and Compliance
- Policy and Framework Development: Design and enforce rules regarding data usage, access control, lineage, and retention.
- Auditability and Traceability: Ensure all data interactions are logged and monitored to support audits, compliance checks, and risk mitigation.
4. Advanced Analytics Integration
- Predictive and Prescriptive Analytics: Use AI/ML to move from descriptive analytics (what happened) to predictive (what will happen) and prescriptive (what to do).
- Business Intelligence Dashboards: Integrate with platforms like Tableau, Power BI, or Looker to visualize KPIs and trends for non-technical stakeholders.
5. Self-Service Enablement
- Democratization of Data: Provide user-friendly tools that empower non-technical users to access, query, and interpret data independently.
- Cross-Departmental Collaboration: Foster a culture where departments can share insights and collaborate without data silos or IT bottlenecks.
Benefits of Data Modernization
Organizations that successfully embrace data modernization experience transformative advantages, including:
- Rapid Access to Real-Time Insights: Shift from reactive decision-making to proactive strategies based on live data analysis.
- Enhanced Customer Experience and Personalization: Use behavioral and transactional data to offer personalized content, offers, and experiences.
- Greater Agility in Responding to Market Changes: Quickly pivot business strategies or adapt operations in response to dynamic market conditions.
- Reduced IT Complexity and Maintenance Costs: Decrease reliance on outdated hardware, manual processes, and custom scripts that require frequent fixes.
- Improved Compliance and Data Governance: Ensure alignment with industry regulations (e.g., GDPR, HIPAA) through automated monitoring and policy enforcement.
Challenges of Data Modernization
Despite its benefits, data modernization is a complex undertaking with several potential challenges:
- Resistance to Change from Internal Teams: Employees accustomed to legacy tools and workflows may resist adopting new platforms or approaches.
- High Upfront Investment and Planning Effort: Requires detailed planning, initial financial investment, and potentially long transition periods.
- Managing Data Sprawl and Interoperability: Ensuring seamless integration across multiple systems, formats, and departments can be technically demanding.
- Need for Skilled Resources and Change Management: Demands experienced data architects, engineers, and a structured change management approach to ensure smooth adoption.
Role of Data Analytics Consulting Services in Data Modernization
Implementing a successful data modernization strategy often necessitates expert guidance. That’s where Data Analytics Consulting Services become invaluable. Their contributions include:
- Strategic Assessment and Roadmap Development: Consultants assess current systems and design tailored modernization blueprints aligned with business goals.
- Technology Selection and Implementation: They help choose the right mix of tools—cloud platforms, data lakes, analytics engines—and assist in implementation.
- Data Governance Frameworks: Experts design governance policies that meet regulatory standards and enhance data quality.
- AI/ML Integration: Specialized teams enable predictive analytics by integrating custom or off-the-shelf AI models into your ecosystem.
- Change Management and Training: Consultants support cultural shifts by educating internal stakeholders, ensuring adoption and long-term success.
Data Migration vs. Data Modernization: A Comparative Analysis
Understanding the difference between data migration and data modernization is critical for organizations embarking on a digital transformation journey. Although the terms are often used interchangeably, they represent distinct strategies with different goals, scopes, and impacts.
The table below presents a side-by-side comparison of key features, followed by a detailed analysis of each element to clarify how these two approaches diverge and complement each other.
Comparative Table: Data Migration vs. Data Modernization
Feature/Aspect | Data Migration | Data Modernization |
Definition | Transfer of data from one environment to another | Comprehensive overhaul of the data strategy and infrastructure |
Primary Goal | Relocate data efficiently and securely | Unlock business value through advanced data capabilities |
Timeframe | Short to medium-term project | Long-term, continuous strategic initiative |
Technologies Involved | ETL tools, data loaders, database replication platforms | Cloud platforms, AI/ML, data lakes, lakehouses, real-time analytics |
Process Complexity | Moderate (focused on technical execution) | High (requires technical, organizational, and cultural transformation) |
End Result | Data is accessible in a new system | Enhanced data usability, agility, scalability, and insight generation |
Cost Impact | One-time investment | Long-term ROI through improved efficiency and innovation |
In-Depth Analysis of Key Features
- Data Migration refers to the movement of data between systems, formats, or environments. This can include transferring data from an on-premises server to the cloud, or from one software platform to another.
- Data Modernization, in contrast, is a holistic transformation. It involves reengineering the data architecture, integrating modern technologies like real-time analytics, and enhancing data governance policies to align with strategic business needs.
Primary Goal
- Migration focuses on relocating data with minimal disruption.
- Modernization aims to unlock business value by making data more accessible and actionable.
Timeframe
- Migration is a short-term project with a clear end once data is moved and validated.
- Modernization is a long-term, evolving journey that adapts to technology and business needs over time.
Technologies Involved
- Data Migration Tools include:
- ETL (Extract, Transform, Load) tools such as Talend, Informatica, and Apache Nifi
- Database replication tools like AWS DMS or Oracle GoldenGate
- Cloud migration platforms such as Azure Migrate or Google Cloud Transfer
- Data Modernization Technologies include:
- Cloud platforms (AWS, Azure, GCP)
- Data Lakes and Lakehouses (Databricks, Snowflake)
- AI/ML frameworks (TensorFlow, PyTorch)
- Real-time analytics tools (Kafka, Apache Flink)
Process Complexity
- Migration is a technical effort focused on data integrity and system compatibility.
- Modernization is a strategic initiative requiring cross-functional collaboration, change management, and alignment with long-term business goals.
End Result
- Data migration moves data to a new system without necessarily improving its usability.
- Data modernization transforms data into a strategic asset, enabling real-time insights and scalable growth.
Cost Impact
- Migration has upfront, short-term costs for tools, infrastructure, and labor.
- Modernization requires higher initial investment but drives long-term ROI through efficiency, better customer experiences, and faster innovation.
By partnering with Data Analytics Consulting Services, businesses can optimize their investment and avoid common pitfalls in both migration and modernization.
The Role of Data Analytics Consulting Services
Why Data Analytics Consulting Services Matter
Both data migration and data modernization are complex undertakings that require precise planning, execution, and quality control. While internal IT departments may possess baseline technical capabilities, they often lack the specialized skills and holistic perspectives that these consulting services bring to the table.
Data Analytics Consulting Services bridge the gap between technical execution and business strategy, ensuring that organizations achieve both operational efficiency and analytical maturity.
Role of Data Analytics Consulting Services in Data Migration
During a data migration project, consulting services provide essential support across the project lifecycle—from initial planning to post-migration audits. Their expertise helps organizations execute seamless migrations with minimal disruption and maximum data fidelity.
Key Contributions in Data Migration:
- Defining Data Migration Roadmaps: Consultants assess the existing infrastructure, identify dependencies, and design a clear, step-by-step migration strategy aligned with business timelines.
- Selecting Suitable Migration Tools and Platforms: With experience across various industries and platforms, consultants recommend tools that ensure speed, reliability, and compatibility with enterprise systems.
- Ensuring Data Integrity and Testing Protocols: Maintaining the accuracy and consistency of data during transfer is crucial. Consulting services implement rigorous testing methodologies to validate data quality.
- Training Internal Teams on New Systems: Smooth adoption of new platforms requires skill development. Consultants conduct training sessions and create documentation to support user onboarding.
- Regulatory and Compliance Management: Particularly in industries like finance and healthcare, consultants ensure that data migration complies with relevant regulations such as GDPR, HIPAA, or SOX.
With the guidance of Data Analytics Consulting Services, organizations experience smoother transitions with fewer operational disruptions and improved post-migration performance.
Role of Data Analytics Consulting Services in Data Modernization
Data modernization is not just a technical initiative—it is a strategic transformation of how data is collected, stored, governed, and analyzed. In such complex scenarios, Data Analytics Consulting Services play a central role in designing, executing, and governing modernization programs.
Key Contributions in Data Modernization:
- Designing Modern Data Architectures: Consultants help build cloud-native, scalable, and secure data ecosystems, including data lakes, warehouses, and streaming pipelines.
- Choosing the Right Cloud Strategy: Whether it’s public, private, or hybrid, consultants assess operational needs and regulatory constraints to recommend the most suitable cloud approach.
- Implementing Governance Frameworks: Modern data governance involves access control, data lineage, auditing, and compliance. Consulting services implement comprehensive frameworks for governance and security.
- Integrating AI/ML and Real-Time Analytics Capabilities: Advanced analytics, powered by artificial intelligence and machine learning, require infrastructure, data pipelines, and algorithmic development—all of which consultants orchestrate.
- Enabling Self-Service and Democratization: Consultants implement user-friendly tools and dashboards that empower non-technical users to derive insights independently, thereby reducing bottlenecks in decision-making.
- Measuring ROI and Success Metrics: One of the most overlooked aspects of modernization is performance measurement. Consultants define and track KPIs to ensure sustained value realization.
Business Outcomes with Consulting Services
The involvement of experienced consultants in your data strategy doesn’t just ensure smoother execution—it also delivers measurable business value. Several industry studies and internal case assessments demonstrate the following outcomes:
Quantifiable Benefits:
- 30–40% Faster Project Delivery: Through optimized planning, tool selection, and best practices, consulting services accelerate migration and modernization timelines.
- Up to 50% Fewer Operational Errors: Expertise in testing, validation, and error handling leads to cleaner implementations with fewer post-deployment issues.
- Greater Alignment with Business Objectives: Consultants help ensure that technical implementations support strategic goals—whether that’s improving customer analytics, enabling AI, or scaling infrastructure.
When to Engage Data Analytics Consulting Services
Organizations should consider engaging consulting services under the following conditions:
- Lack of in-house expertise in modern cloud or analytics platforms
- Large-scale migration from legacy databases or monolithic applications
- Need for a comprehensive data governance strategy
- Goals to implement real-time analytics or predictive modeling
- Tight timelines or mission-critical systems that can’t afford migration risks
- Challenges in aligning data strategy with overall business growth
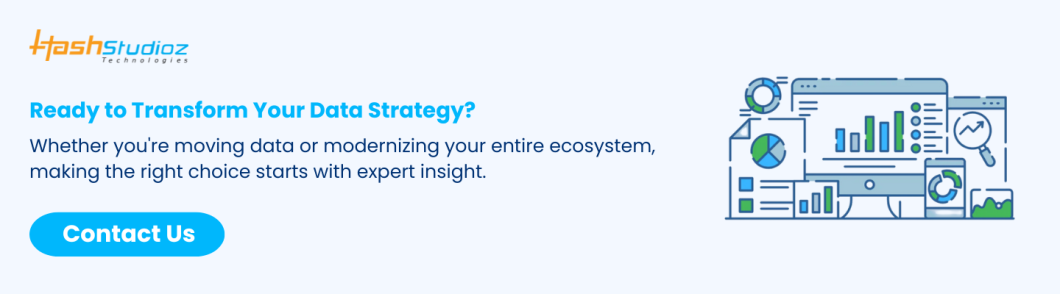
Conclusion
Data migration and data modernization serve different but complementary purposes in a company’s data strategy. While data migration ensures that data is efficiently relocated to newer environments, data modernization seeks to transform the very approach to data utilization for better innovation, performance, and growth.
Businesses must clearly define their goals and engage the right expertise—especially through Data Analytics Consulting Services—to implement these strategies successfully. With the right approach, organizations can reduce technical debt, boost operational efficiency, and drive more informed decisions.
FAQs
1. Is data migration necessary before data modernization?
In most cases, yes. Data migration lays the groundwork by moving data from legacy systems to modern platforms, enabling further modernization steps.
2. How long does a data modernization project take?
It depends on the organization’s size, complexity, and objectives. Projects can range from several months to over a year.
3. What are the risks of not modernizing data?
Staying with legacy systems can lead to:
- Inability to scale
- Increased costs
- Lack of agility and innovation
- Poor customer experiences
4. Can Data Analytics Consulting Services help small businesses?
Absolutely. Consulting services offer scalable solutions tailored to any business size, helping even small enterprises unlock the value of their data.
5. What tools are commonly used in data migration and modernization?
- Migration Tools: AWS DMS, Azure Migrate, Informatica, Talend
- Modernization Tools: Snowflake, Databricks, Apache Kafka, Power BI, Tableau