Organizations across industries are generating data at an unprecedented pace. From customer behavior to supply chain metrics, data is flowing in from structured, semi-structured, and unstructured sources. Managing this deluge of data efficiently and converting it into business intelligence is no small feat. This is where Data Lake Consulting Services come into play.
Whether you’re a startup looking to build a modern data architecture or an enterprise aiming to optimize your current analytics infrastructure, understanding the scope, benefits, and strategic impact of data lake consulting is essential.
- The global data lake market is expected to reach $24.7 billion by 2027 with a CAGR of over 20%.
- Over 60% of enterprises are adopting hybrid data lake architectures for analytics and ML workloads.
- Businesses leveraging Data Lake Consulting Services report up to 40% faster deployment and 30–50% reduction in data infrastructure costs.
Table of Contents
- What Are Data Lake Consulting Services?
- Key Components of a Data Lake
- Benefits of Data Lake Consulting Services
- Challenges in Implementing Data Lake Solutions
- How Data Lake Consulting Services Solve These Challenges
- Common Use Cases of Data Lake Consulting Services
- Data Lake vs. Data Warehouse: Understanding the Difference
- Key Technologies in Data Lake Implementation
- Best Practices Recommended by Data Lake Consultants
- Choosing the Right Data Lake Consulting Partner
- Future Trends in Data Lake Consulting Services
- Conclusion
- FAQs
What Are Data Lake Consulting Services?
Core Components of Data Lake Consulting Services
Data Lake Consulting Services typically encompass the following critical areas:
- Architecture Design and Planning: Consultants assess business goals, current data infrastructure, and scalability needs to develop a tailored data lake architecture. This includes deciding on storage layers, data zones (raw, refined, curated), and logical/physical schema design.
- Technology Selection: Selection of appropriate technologies is a crucial step. This may involve choosing between cloud platforms like AWS S3, Azure Data Lake Storage, or Google Cloud Storage, as well as related tools such as Apache Hadoop, Apache Spark, or Databricks.
- Integration of Data Sources: Consultants help organizations integrate disparate data sources, including relational databases, SaaS platforms, real-time streams, IoT devices, and on-premises systems, into a unified data lake.
- Governance and Security Framework: Establishing robust governance frameworks ensures that the data lake remains compliant with internal policies and external regulations (e.g., GDPR, HIPAA). Security involves identity and access management, encryption, auditing, and data lineage tracking.
- Data Ingestion and ETL Pipelines: Specialists design scalable pipelines to ingest data in batch or real-time, transforming and preparing it for downstream analytics, machine learning, or visualization use cases.
- Query Performance Optimization: Consultants fine-tune performance by implementing partitioning strategies, optimizing metadata catalogs, caching frequently used queries, and leveraging columnar formats like Parquet and ORC.
- Training and Support: Teams are provided with hands-on training, documentation, and ongoing support to ensure successful adoption and long-term sustainability of the data lake solution.
Strategic Role of Data Lake Consultants
Data Lake consultants are more than technical enablers; they are strategic partners who help align data lake initiatives with business objectives. By drawing on domain knowledge and best practices, these experts:
- Reduce the time and risk associated with building complex data infrastructures
- Ensure cost-efficient and scalable solutions that support future data growth
- Promote best practices in data governance, quality, and analytics readiness
Key Components of a Data Lake
Understanding the core components of a data lake helps organizations grasp why consulting services are crucial. A typical data lake includes:
- Data Ingestion Layer: Captures data from various sources like IoT devices, social media, CRMs, and ERP systems.
- Storage Layer: Often built on object storage like Amazon S3 or Azure Data Lake Storage, optimized for scalability and cost.
- Processing Layer: Tools like Apache Spark, AWS Glue, or Azure Data Factory are used to transform raw data.
- Metadata & Cataloging: Ensures discoverability of datasets via tools like AWS Glue Data Catalog or Apache Atlas.
- Security & Governance Layer: Manages access controls, encryption, and compliance frameworks.
- Analytics & Visualization: Enables end-users to extract insights using BI tools like Looker, Tableau, or Power BI.
Benefits of Data Lake Consulting Services
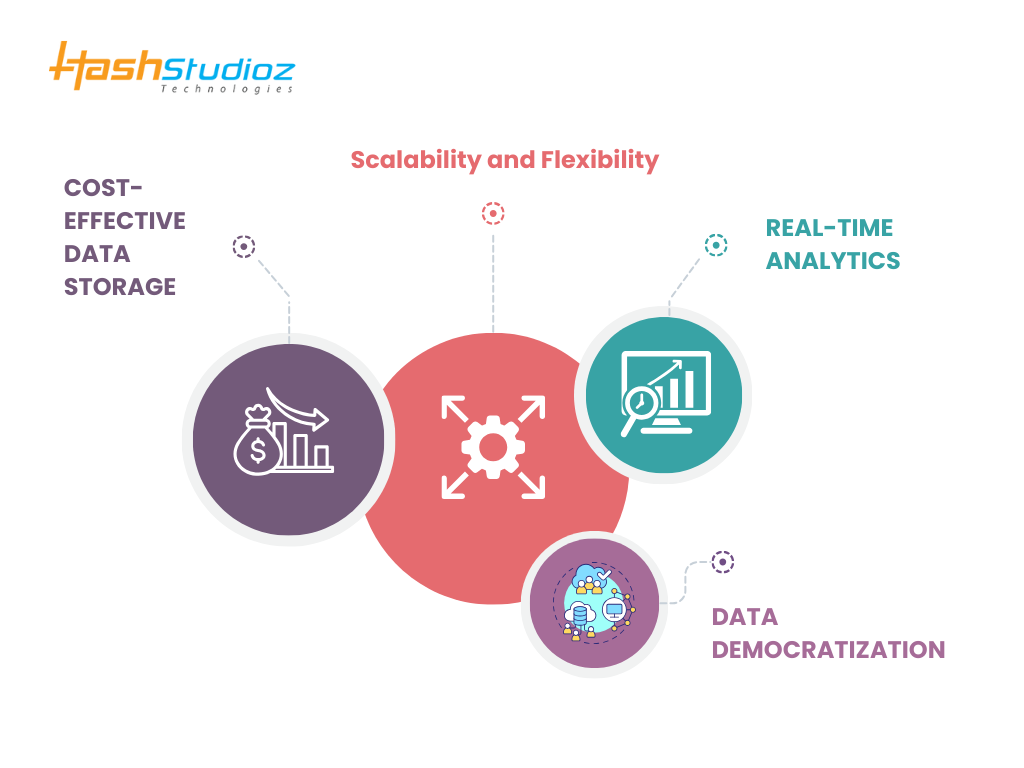
a. Cost-Effective Data Storage
- Storing massive volumes of data in traditional databases is expensive.
- Data lakes, especially on cloud infrastructure, provide affordable object-based storage.
- Consultants help design tiered storage policies for cost optimization.
b. Scalability and Flexibility
- Data lake architectures are inherently scalable.
- Consultants help architect elastic environments that grow with your data needs.
- Flexibility to store structured, semi-structured, and unstructured data.
c. Real-Time Analytics
- Consultants design pipelines that support near real-time data ingestion and processing.
- Empower business users with dashboards and alerts using real-time datasets.
d. Data Democratization
- Centralized data access across teams leads to improved collaboration.
- Consultants implement role-based access controls to ensure secure democratization.
- Enhances self-service BI capabilities for data analysts and business users.
The Role of Data Lake Consulting in Enabling AI and ML Solutions
Challenges in Implementing Data Lake Solutions
While data lakes offer a multitude of benefits, their implementation is not without obstacles.
a. Data Governance
- Without governance, data lakes can turn into data swamps.
- Lack of metadata and data cataloging leads to untraceable datasets.
b. Security Concerns
- Data lakes house sensitive data, making them targets for cyber threats.
- Improper configuration leads to data breaches and non-compliance.
c. Data Quality and Lineage
- Unstructured data can be inconsistent, incomplete, or duplicated.
- Tracking data lineage across pipelines is complex.
d. Skills Gap
- Lack of in-house expertise in big data frameworks and tools.
- Poor understanding of cloud platforms and orchestration tools.
How Data Lake Consulting Services Solve These Challenges
Governance Frameworks
- Implement metadata management tools (Apache Atlas, AWS Glue Catalog).
- Define naming conventions, taxonomy, and lifecycle policies.
Security Implementation
- Enable encryption at rest and in transit.
- Use IAM roles and policies for granular access control.
Data Quality Monitoring
- Set up validation rules and cleansing pipelines.
- Introduce lineage tracking tools like DataHub or OpenLineage.
Skills Augmentation
- Provide staff training and upskilling programs.
- Offer long-term support and managed services.
ETL vs. ELT: Choosing the Right Data Ingestion Strategy with Data Lake Consulting Services
Common Use Cases of Data Lake Consulting Services
Data Lake Consulting Services help organizations across industries centralize, manage, and analyze vast volumes of data. Below are key industry-specific applications:
a. E-commerce
- Customer Behavior Analysis: Track clickstreams and purchase patterns for segmentation and personalization.
- Inventory Optimization: Use real-time sales and supply data to avoid overstock or shortages.
- Personalized Marketing: Deliver tailored campaigns based on user behavior and preferences.
b. Healthcare
- Patient Data Aggregation: Integrate EHRs, lab results, and clinical notes into a unified patient profile.
- IoT Monitoring: Stream data from wearables for real-time health tracking.
- Predictive Diagnostics: Use historical and real-time data to detect disease risks.
c. Financial Services
- Fraud Detection: Implement ML models to flag unusual activity.
- Risk & Compliance: Centralize regulatory and transaction data for audits.
- Credit Scoring: Use alternative data sources to expand access to credit.
d. Manufacturing
- IoT Sensor Data: Monitor machines for performance and anomalies.
- Predictive Maintenance: Prevent breakdowns by analyzing usage patterns.
- Quality Control: Analyze defect trends to improve product standards.
e. IoT & Smart Cities
- Traffic Telemetry: Optimize traffic flow using real-time sensor data.
- Energy Monitoring: Track and forecast power usage from smart meters.
- Environmental Tracking: Monitor air and water quality using sensor networks.
Data Lake vs. Data Warehouse: Understanding the Difference
Feature | Data Lake | Data Warehouse |
Data Type | Structured, semi-structured, unstructured | Structured only |
Storage Cost | Lower due to object storage | Higher due to specialized storage |
Schema | Schema-on-read | Schema-on-write |
Performance | High for large-scale analytics | High for structured queries |
Use Cases | ML, AI, real-time analytics | BI, reporting, dashboarding |
Data Lake Consulting Services often complement data warehouses by building hybrid architectures for different analytical needs.
Key Technologies in Data Lake Implementation
Implementing a data lake involves integrating various technologies that support storage, processing, governance, and visualization. Data Lake Consulting Services guide organizations in selecting and orchestrating the right tools to ensure performance, scalability, and compliance.
1. Storage
Modern data lakes rely on scalable, cloud-based object storage to handle structured, semi-structured, and unstructured data.
- AWS S3: Highly scalable and durable object storage service.
- Azure Data Lake Storage: Designed for big data analytics with hierarchical namespace.
- Google Cloud Storage: Unified storage for analytics and machine learning workflows.
2. Processing Engines
These engines process and transform large volumes of data stored in the lake.
- Apache Spark: Distributed processing engine for batch and real-time data.
- Presto: High-performance SQL query engine for interactive analytics.
- Apache Hive: SQL-based engine for querying large datasets.
- AWS Glue: Serverless ETL tool that automates data transformation.
3. Orchestration Tools
These tools schedule and manage complex data workflows.
- Apache Airflow: Workflow automation and scheduling platform.
- Azure Data Factory: Cloud-based ETL and pipeline orchestration tool.
- AWS Step Functions: Serverless orchestration for microservices and workflows.
4. Metadata Management
Metadata tools are essential for governance, lineage tracking, and data discovery.
- Apache Atlas: Provides data classification, lineage, and security.
- Amundsen: Metadata catalog for data discovery and collaboration.
- AWS Glue Catalog: Central metadata repository for AWS analytics services.
5. Security Tools
Security frameworks protect sensitive data and enforce access controls.
- AWS IAM: Identity and access management for user permissions.
- Azure RBAC: Role-based access control for data lake operations.
- Encryption Libraries: Ensure data is encrypted at rest and in transit.
6. Visualization Tools
These tools convert data into actionable insights for decision-makers.
- Power BI: Microsoft’s business intelligence platform for interactive dashboards.
- Looker Studio: Google’s data visualization platform for real-time insights.
- Tableau: Widely used analytics platform with powerful visualization capabilities.
Data Warehouse vs. Data Lake vs. Lakehouse: Which Fix is Best?
Best Practices Recommended by Data Lake Consultants
Based on experience across industries, Data Lake Consulting Services emphasize the following best practices to maximize ROI and ensure sustainable implementation:
1. Start Small, Scale Fast: Begin with a limited-scope pilot project to prove value, then expand based on performance and user feedback.
2. Define Data Ownership: Assign data stewards responsible for maintaining data quality, security, and compliance across departments.
3. Automate Ingestion Pipelines: Use scalable ETL/ELT tools to automate data ingestion from various sources, minimizing manual errors.
4. Implement Logging and Monitoring: Establish robust logging mechanisms and monitor data pipelines to detect failures, latency, or storage inefficiencies.
5. Conduct Regular Audits : Periodically review access logs, data quality metrics, and compliance status to ensure alignment with governance policies.
Choosing the Right Data Lake Consulting Partner
Selecting the right provider of Data Lake Consulting Services ensures long-term success. Key criteria include:
- Cloud Expertise: Proven skills in AWS, Azure, and Google Cloud.
- Industry Knowledge: Experience in domains like healthcare, finance, and retail.
- Compliance Focus: Familiarity with regulations such as GDPR, HIPAA, and CCPA.
- Flexible Engagement Models: Offers full implementation or staff augmentation.
- Client References: Strong portfolio with real-world success stories.
Future Trends in Data Lake Consulting Services
As data ecosystems evolve, Data Lake Consulting Services are rapidly adapting to support next-generation architectures and technologies. The following trends highlight the future direction of this domain:
1. Lakehouse Architecture: This hybrid model merges the flexibility of data lakes with the performance of data warehouses. Benefits include:
- Unified storage layer
- Support for ACID transactions
- Simpler analytics workflows
Consultants will increasingly help businesses transition to platforms like Databricks Lakehouse or Delta Lake.
2. AI-Powered Governance: Machine learning will play a bigger role in automating:
- Data cataloging and tagging
- PII detection and classification
- Policy enforcement based on usage patterns
Expect AI-driven platforms to reduce manual data governance effort.
3. Serverless Data Lakes: With the rise of tools like AWS Athena, BigQuery, and Azure Synapse Serverless, the future points toward:
- On-demand, pay-per-query models
- No infrastructure management
- Faster time-to-insight
Consultants will guide organizations in migrating to cost-efficient, elastic analytics environments.
4. Real-Time Data Lakes: More businesses require real-time streaming capabilities to power:
- Fraud detection
- Customer engagement personalization
- Operational dashboards
Apache Kafka, Apache Flink, and Spark Streaming will be key technologies, with consulting firms designing architectures that reduce latency.
5. Data Mesh Integration: This modern approach promotes decentralized data ownership, enabling:
- Domain-specific data products
- Self-serve infrastructure
- Better data quality through accountability
Data Lake Consulting Services will increasingly include guidance on implementing data mesh principles within enterprise architectures.
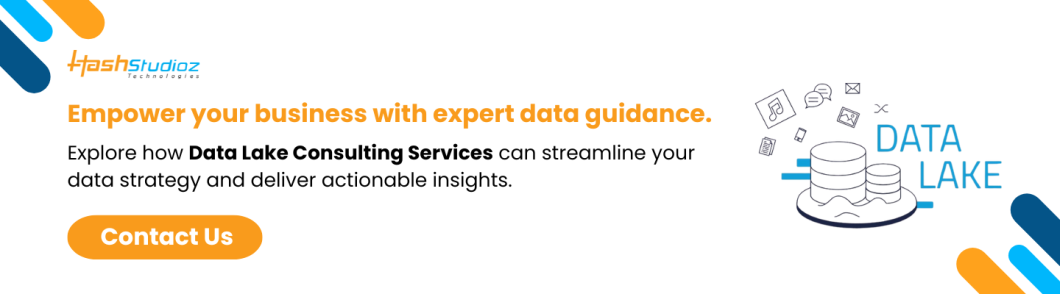
Conclusion
As data complexity continues to grow, the role of Data Lake Consulting Services becomes increasingly indispensable. From designing scalable architectures to overcoming governance and security hurdles, consulting partners empower businesses to extract actionable insights from diverse data sources. By embracing these services, organizations unlock the true potential of their data assets and position themselves for sustained competitive advantage.
Whether you’re in healthcare, e-commerce, finance, or manufacturing, the strategic implementation of data lakes—guided by expert consultants—can transform your decision-making and innovation capabilities.
FAQs
Q1: What is the main purpose of Data Lake Consulting Services?
To help businesses design, build, and maintain scalable, secure, and efficient data lakes tailored to their analytical needs.
Q2: How are data lakes different from data warehouses?
Data lakes handle structured and unstructured data using schema-on-read, while data warehouses are optimized for structured data and schema-on-write.
Q3: Can small businesses benefit from Data Lake Consulting Services?
Absolutely. Consultants can design cost-effective, scalable solutions even for startups and SMEs using cloud-native tools.
Q4: What industries benefit the most from data lakes?
E-commerce, healthcare, finance, manufacturing, and IoT are major adopters due to the need for diverse data analysis.
Q5: How long does it take to implement a data lake?
Depending on complexity, initial implementation can range from a few weeks (for MVPs) to several months (for enterprise-scale systems).