Big Data Lakes are integral to modern data architectures, enabling organizations to store vast amounts of raw, unstructured, and structured data at scale. However, choosing the right storage format for your Big Data Lake is crucial for ensuring optimal performance, consistency, and scalability. In this blog, we’ll explore three popular open-source formats for managing large-scale data lakes: Delta Lake, Apache Iceberg, and Apache Hudi. By the end of this post, you’ll have a clearer understanding of each format’s features and differences, helping you decide which one fits your use case best.
Table of Contents
- Overview of Big Data Lakes
- Importance of Choosing the Right Format
- What is Delta Lake?
- What is Apache Iceberg?
- What is Apache Hudi?
- Delta Lake vs. Apache Iceberg vs. Hudi: Key Differences
- How to Choose the Right Format for Your Big Data Lake
- Harnessing Big Data Lakes: Why HashStudioz Stands Out in Data Analytics
- Conclusion
Overview of Big Data Lakes
Big Data Lakes are repositories designed to store massive amounts of raw data in its native format. Unlike traditional databases, they can handle data in a variety of formats such as structured, semi-structured, and unstructured data. As businesses continue to generate vast amounts of data, it’s essential to choose the correct storage format to manage this data efficiently.
Importance of Choosing the Right Format
Data lakes provide flexibility, but with great flexibility comes complexity. The right format ensures that your Big Data Lake scales efficiently, supports ACID transactions, handles schema changes gracefully, and integrates seamlessly with data processing tools. The wrong choice can lead to performance bottlenecks, data inconsistencies, and scalability challenges.
What is Delta Lake?
Delta Lake is an open-source storage layer that brings reliability, performance, and scalability to data lakes. Built on top of Apache Spark and Parquet, Delta Lake enhances the capabilities of traditional data lakes by providing ACID (Atomicity, Consistency, Isolation, Durability) transaction support, schema enforcement, and time travel features. It is designed to handle both batch and streaming data, making it a versatile solution for modern data engineering and analytics.
Key Features of Delta Lake
Delta Lake is an open-source storage layer built on top of Apache Spark and Parquet. It brings ACID transaction guarantees to Big Data workloads, which is a crucial feature when managing large datasets. Delta Lake supports schema enforcement, schema evolution, and time travel (versioning of data), allowing for strong consistency and Security in Big Data Lake.
- ACID Transactions: Ensures data consistency during read/write operations.
- Schema Enforcement & Evolution: Allows for changes in the schema over time without disrupting data integrity.
- Time Travel: Enables querying historical data by maintaining multiple versions of datasets.
Use Cases for Delta Lake
Delta Lake is well-suited for applications that require reliable, consistent, and scalable data storage. Common use cases include:
- ETL (Extract, Transform, Load) processes
- Streaming and batch data processing
- Machine learning and analytics workflows
What is Apache Iceberg?
Apache Iceberg is an open-source table format designed for large-scale data lakes. It provides a high-performance solution for managing petabyte-scale datasets, enabling organizations to efficiently store, process, and analyze vast amounts of data. Iceberg was developed to address the challenges associated with traditional data lake formats, such as performance issues, schema evolution, and data consistency.
Key Features of Apache Iceberg
Apache Iceberg is a high-performance format for large-scale tables in Big Data Lakes. It is designed to handle petabyte-scale datasets and supports features like schema evolution, partitioning, and versioning. Iceberg also focuses on providing compatibility with many data processing engines such as Apache Spark, Hive, Flink, and Presto.
- Schema Evolution: Allows for easy changes in data structure without affecting existing data.
- Partitioning: Provides more flexible and efficient data partitioning strategies.
- Atomicity and Consistency: Ensures data integrity through versioned snapshots and atomic commit protocols.
Use Cases for Apache Iceberg
Apache Iceberg excels in scenarios where high performance and flexibility are key, particularly with petabyte-scale data:
- Cloud-native Big Data Lakes
- Analytical and data warehousing applications
- Multi-engine data processing environments
What is Apache Hudi?
Apache Hudi (Hadoop Upserts Deletes and Incrementals) is an open-source data management framework designed for large-scale data lakes. It provides capabilities for managing both batch and streaming data, enabling efficient data ingestion, storage, and processing. Hudi is particularly well-suited for scenarios that require real-time data updates, incremental processing, and ACID (Atomicity, Consistency, Isolation, Durability) transactions.
Key Features of Apache Hudi
Apache Hudi is a distributed data lake storage format that supports both batch and stream processing. It is designed to enable efficient incremental processing and upsert operations in Big Data Lakes, with features like data versioning, ACID transactions, and real-time stream processing.
- Real-time Data Processing: Efficient support for upserts and incremental processing.
- ACID Transactions: Ensures atomicity of read/write operations for consistency.
- Data Versioning: Supports storing multiple versions of data for historical analysis.
Use Cases for Apache Hudi
Apache Hudi is ideal for applications that need to handle real-time data ingestion and processing:
- Data pipelines with real-time updates
- Incremental data processing for analytics
- Applications that require low-latency data updates
Delta Lake vs. Apache Iceberg vs. Hudi: Key Differences
Feature | Delta Lake | Apache Iceberg | Apache Hudi |
Data Model & Structure | Built on Parquet, integrates with Spark, uses log-based architecture for transaction consistency. | Focuses on tables, with advanced partitioning and indexing for high performance. | Combines columnar and row-level storage, supports both batch and streaming. |
Performance & Scalability | Good performance for batch and streaming workloads via Apache Spark. | Excels in scalability, especially for petabyte-scale tables. | Strong in real-time and incremental processing, low-latency updates and upserts. |
Consistency & ACID Transactions | Full ACID transaction support, ensures consistency with transaction log. | Strong consistency and atomic operations, safe writes even in high concurrency. | Supports ACID transactions, designed for real-time updates and incremental processing. |
Schema Evolution & Management | Automatic schema evolution and enforcement for data structure changes. | Flexible schema evolution supports complex changes without breaking pipelines. | Schema evolution and large-scale data updates via upsert capabilities. |
Support for Streaming Data | Native support for both batch and streaming data processing via Apache Spark Structured Streaming. | Primarily batch processing, but supports streaming via Flink and Spark. | Strong focus on streaming data, supports real-time updates and incremental ingestion. |
Integration with Other Tools & Ecosystems | Tight integration with the Spark ecosystem, supports other frameworks. | Cross-engine compatibility (Apache Spark, Hive, Flink, Presto). | Best known for Apache Spark integration, also works with Hive and Presto. |
How to Choose the Right Format for Your Big Data Lake
When selecting the appropriate format for your Big Data Lake, it’s crucial to consider several factors that align with your Big Data Analytics Services needs:
1. Data Size
Apache Iceberg is ideal for handling massive petabyte-scale datasets, while Delta Lake and Apache Hudi are well-suited for both batch and streaming data scenarios.
2. Use Case
Delta Lake supports ACID transactions, Iceberg excels in table-based partitioning and scalability, and Hudi handles real-time incremental processing.
3. Performance
If your requirements include high throughput and low-latency updates, both Hudi and Iceberg are likely to meet your needs more effectively.
4. Ecosystem Compatibility
Consider the data processing tools you are utilizing (such as Spark, Flink, Hive, etc.) when selecting a format, as compatibility can significantly impact the efficiency of your Big Data Analytics Services.
Harnessing Big Data Lakes: Why HashStudioz Stands Out in Data Analytics
HashStudioz is a leading Data Analytics Services Company known for delivering tailored solutions that enhance data management and analytics capabilities. Their expertise in building scalable and flexible Big Data Lakes ensures businesses can efficiently store, process, and analyze vast amounts of data, driving better decision-making and insights.
1. Expertise in Data Management
HashStudioz has a team of skilled professionals with extensive experience in managing and optimizing Big Data Lakes, ensuring that your data is handled efficiently.
2. Custom Solutions
They offer tailored solutions that cater to the specific needs of your business, allowing for a more personalized approach to data storage and analytics.
3. Integration with Advanced Technologies
HashStudioz seamlessly integrates with leading technologies such as Google Cloud and Azure, providing robust analytics capabilities and real-time insights.
4. Scalability
Their Big Data Lake solutions scale with your business, accommodating growing data volumes without compromising performance.
5. Real-Time Analytics
HashStudioz enables businesses to leverage real-time data analytics, allowing for quicker decision-making and enhanced operational efficiency.
6. Data Security and Compliance
They prioritize data security, protecting your sensitive information and ensuring compliance with industry standards.
7. Support for Diverse Data Types
HashStudioz can manage various data types, including structured, semi-structured, and unstructured data, making it easier to harness the full potential of your data assets.
8. Proven Track Record
With a history of successful implementations, HashStudioz has established itself as a trusted partner for organizations looking to enhance their data capabilities.
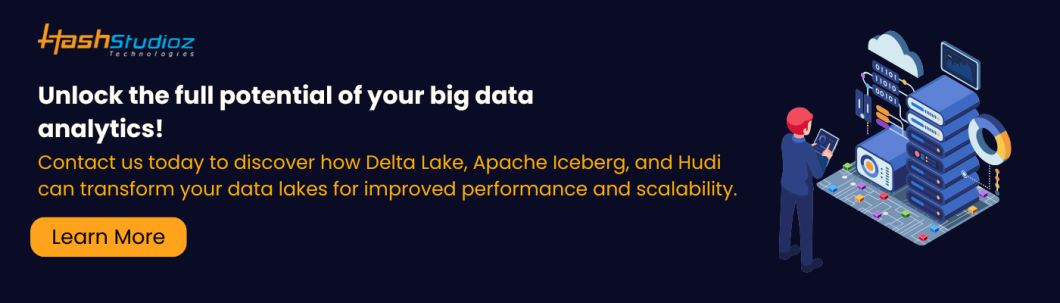
Conclusion
In summary, each of these formats—Delta Lake, Apache Iceberg, and Apache Hudi—offers unique advantages depending on your Big Data Lake needs:
- Delta Lake: Best for ACID transactions and tight integration with Spark.
- Apache Iceberg: Suited for large-scale Big Data Lakes with flexible schema evolution and scalability.
- Apache Hudi: Ideal for real-time data processing and incremental updates.
Carefully assess your Big Data Lake’s requirements, including scale, performance, and processing needs, to choose the format that best aligns with your goals.