In the modern, highly competitive retail landscape, understanding customer needs and preferences has become essential. Retailers are utilizing data analytics services to derive actionable insights from extensive datasets, empowering them to provide personalized and outstanding customer experiences. This article delves into how retailers can strategically leverage data analytics to enhance their customer experience and achieve sustained business growth.
- 80% of customers are more likely to buy from retailers offering personalized experiences.
- 95% of retailers report that data analytics improves decision-making.
- Retailers leveraging analytics see a 30% increase in customer retention rates.
Table of Contents
- The Role of Data Analytics in Modern Retail
- Benefits of Using Data Analytics in Retail
- Key Areas Where Data Analytics Can Improve Customer Experience
- How to Implement Data Analytics in Retail
- Challenges in Using Data Analytics for Retail
- The Future of Retail Analytics in Enhancing Customer Experience
- 1. Hyper-Personalization with AI and Machine Learning
- 2. Seamless Omnichannel Experiences
- 3. AR and VR Integration
- 4. Predictive Analytics for Demand and Stock Optimization
- 5. Advanced Customer Support Automation
- 6. Ethical and Transparent Data Practices
- 7. Real-Time Analytics for Instant Feedback
- 8. Enhanced Sustainability
- Conclusion
- FAQs
The Role of Data Analytics in Modern Retail
Data analytics serves as a cornerstone in modern retail, transforming raw data into actionable insights that drive decision-making and innovation. It empowers retailers to delve deeply into customer preferences, purchasing patterns, and emerging market trends, enabling them to stay ahead of the competition.
By leveraging data analytics services, retailers can unlock significant benefits, including:
- Enhanced Customer Satisfaction: Delivering personalized experiences that meet individual needs and expectations.
- Streamlined Operations: Optimizing inventory, supply chain management, and store efficiency.
- Increased Profitability: Identifying revenue opportunities, reducing waste, and boosting sales through targeted strategies.
Benefits of Using Data Analytics in Retail
Retailers who incorporate data analytics into their operations experience a wide array of benefits, including:
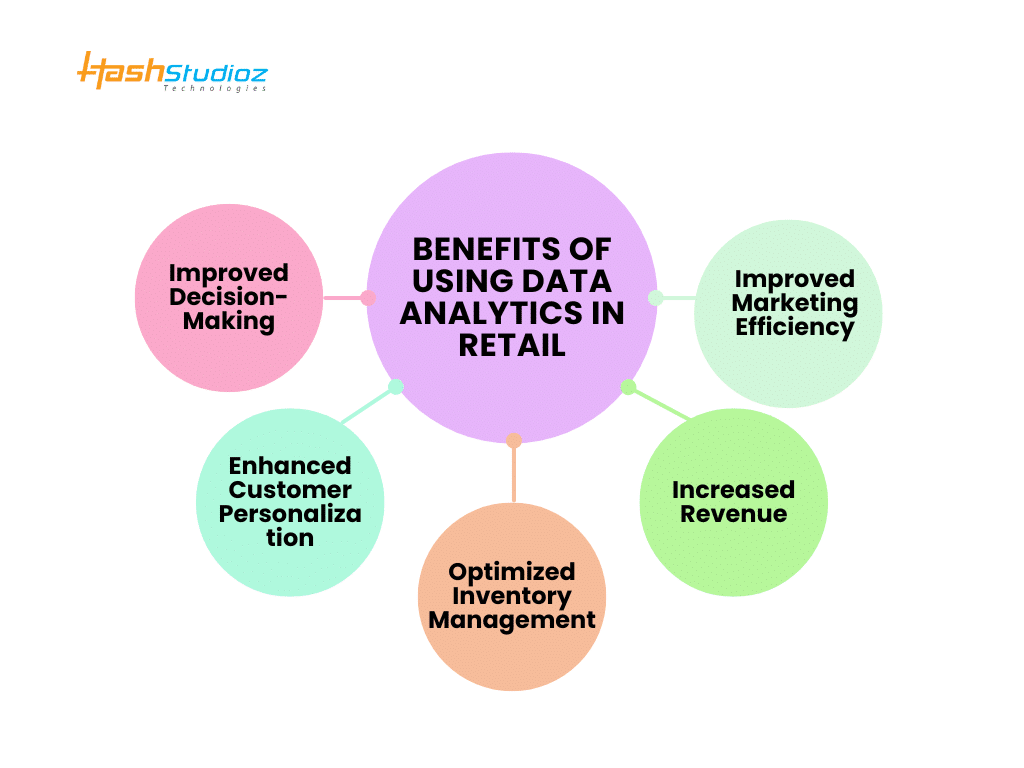
1. Improved Decision-Making: Analytics enables retailers to make informed, data-driven decisions, reducing reliance on guesswork and enhancing strategic planning.
2. Enhanced Customer Personalization: By understanding customer preferences and behaviors, retailers can deliver tailored recommendations, improving satisfaction and loyalty.
3. Optimized Inventory Management: With precise demand forecasting, retailers can avoid the pitfalls of overstocking or understocking, ensuring the right products are always available.
4. Increased Revenue: Providing exceptional, personalized customer experiences leads to higher sales, increased customer retention, and long-term profitability.
5. Improved Marketing Efficiency: Data analytics helps retailers identify the most effective marketing channels and campaigns, allowing for better allocation of budgets and resources while maximizing ROI.
Key Areas Where Data Analytics Can Improve Customer Experience
1. Personalized Recommendations
Personalization is at the core of modern retail strategies, ensuring customers feel valued and understood. Using data analytics:
- Purchase and Browsing Analysis: Retailers can evaluate customer purchase histories and browsing behaviors to recommend products that match their interests.
- Predictive Algorithms: Machine learning algorithms can anticipate what products a customer is most likely to buy.
- Engagement Boost: Personalized emails, product recommendations, and exclusive offers can enhance customer satisfaction and loyalty.
Example: A customer who frequently shops for fitness gear may receive recommendations for related products like running shoes or gym equipment.
2. Inventory Management
Efficient inventory management ensures customers find what they need without delays or stockouts. Data analytics helps by:
- Demand Forecasting: Predicting future demand to ensure optimal stock levels.
- Real-Time Tracking: Monitoring inventory in real time to avoid shortages or overstocking.
- Seasonal Insights: Analyzing past data to prepare for seasonal trends and spikes.
By aligning stock levels with customer demand, retailers minimize costs while maximizing availability.
3. Predictive Analytics for Customer Behavior
Predictive analytics empowers retailers to anticipate and act on customer needs, fostering proactive strategies:
- Churn Prediction: Identifying at-risk customers and implementing retention strategies like exclusive offers or personalized support.
- Lifetime Value Estimation: Assessing a customer’s long-term value to prioritize high-value relationships.
- Behavioral Insights: Understanding customer interactions across various channels to refine the overall shopping experience.
Example: A retailer might notice declining engagement from a loyal customer and offer a special discount to re-engage them.
4. Pricing Optimization
Dynamic pricing strategies powered by data analytics ensure competitiveness and profitability:
- Competitor Analysis: Adjusting prices based on competitor activity to maintain market share.
- Customer Willingness to Pay: Identifying price ranges that align with customer expectations.
- Promotional Effectiveness: Analyzing the impact of discounts and offers to understand what drives sales.
Data-driven pricing strategies help retailers strike a balance between affordability and profit margins.
5. Customer Support Enhancement
Data analytics revolutionizes customer support by offering actionable insights to improve service quality:
- Sentiment Analysis: Gauging customer satisfaction from reviews, social media, and feedback surveys.
- Chatbot Optimization: Training AI-driven chatbots with data to handle common queries efficiently and accurately.
- Support Metrics: Evaluating response times, issue resolution rates, and customer satisfaction scores to identify areas for improvement.
Example: By analyzing feedback, a retailer can identify recurring complaints and implement changes to prevent future issues.
6. Location-Based Marketing
With the widespread use of mobile devices, location-based marketing has become a vital strategy for retailers. Data analytics enables:
- Geofencing: Sending targeted promotions to customers when they are near a store, driving foot traffic.
- Store Performance Analysis: Understanding foot traffic patterns to optimize store layouts and staffing.
- Localized Campaigns: Crafting region-specific promotions based on local customer preferences and trends.
Example: A retailer might send a notification about a flash sale to a customer walking near their store.
How to Implement Data Analytics in Retail
Implementing data analytics in retail requires a structured approach to ensure success and maximize its benefits. Here are the key steps:
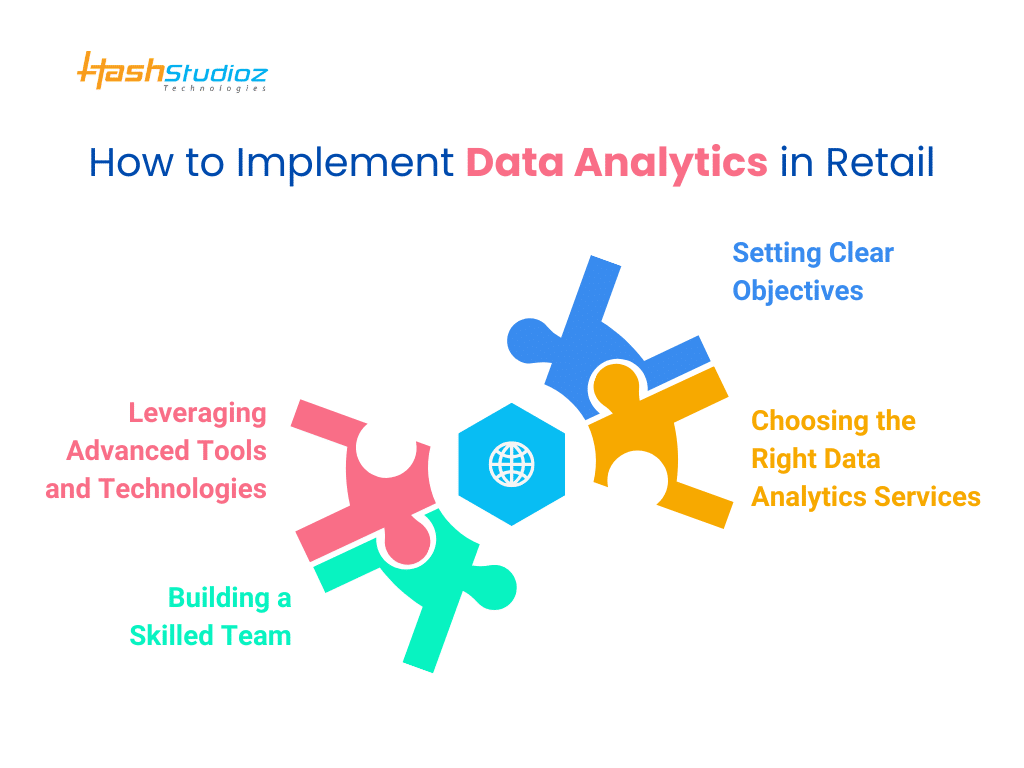
1. Setting Clear Objectives
Before diving into data analytics, retailers must define their goals. Clear objectives guide the entire analytics process and help measure success. Examples of goals include:
- Enhancing Customer Retention: Identifying at-risk customers and implementing strategies to keep them engaged.
- Increasing Average Order Value: Offering personalized upselling or cross-selling opportunities.
- Improving Inventory Turnover Rates: Ensuring the right products are available at the right time to minimize overstock and stockouts.
A well-defined goal ensures that data analytics efforts remain aligned with business priorities.
2. Choosing the Right Data Analytics Services
Selecting a reliable data analytics service provider is crucial for effective implementation. Factors to consider include:
- Expertise in Retail Analytics: Ensure the provider has experience in addressing challenges specific to the retail sector.
- Availability of Advanced Tools and Technologies: Look for providers offering cutting-edge solutions like predictive analytics and real-time reporting.
- Proven Track Record of Success: Review case studies or testimonials to validate the provider’s ability to deliver results.
A competent service provider can significantly ease the implementation process and ensure high-quality outcomes.
3. Building a Skilled Team
The success of any data analytics initiative depends on having the right team in place. Essential roles include:
- Data Scientists: Experts who can analyze and interpret complex datasets to uncover patterns and trends.
- Business Analysts: Professionals who can translate analytical insights into actionable business strategies.
- IT Professionals: Specialists who manage the technical infrastructure, ensuring secure and efficient data collection, storage, and processing.
A collaborative team with diverse expertise ensures a seamless implementation process.
4. Leveraging Advanced Tools and Technologies
Retailers must invest in modern tools and platforms to support their data analytics efforts. Recommended technologies include:
- Customer Relationship Management (CRM) Systems: For tracking customer interactions and maintaining detailed purchase histories.
- Business Intelligence (BI) Tools: To visualize data and generate actionable insights.
- Artificial Intelligence (AI) and Machine Learning (ML) Platforms: To enable predictive analytics and automated decision-making.
These tools not only simplify data analysis but also enhance the accuracy and speed of decision-making.
Challenges in Using Data Analytics for Retail
While data analytics offers transformative benefits, implementing it in retail is not without its challenges. Retailers must navigate several obstacles to unlock its full potential.
1. Data Security and Privacy Concerns
With growing concerns about data breaches and misuse, retailers must adhere to stringent data protection regulations like GDPR or CCPA. This involves:
- Securing Data: Encrypting sensitive customer information and storing it securely to prevent unauthorized access.
- Customer Consent: Ensuring explicit consent is obtained from customers before collecting their data.
- Regulatory Compliance: Regularly auditing data practices to ensure compliance with evolving regulations.
Failing to address privacy concerns can lead to reputational damage and financial penalties.
2. Integration with Existing Systems
Retailers often face difficulties in integrating modern analytics platforms with outdated legacy systems. Common solutions include:
- Migrating to Cloud-Based Platforms: Cloud solutions offer scalability and seamless integration capabilities.
- Using APIs: Application Programming Interfaces (APIs) can bridge the gap between disparate systems, enabling data flow across platforms.
Proper planning and phased integration strategies can help retailers overcome these technical hurdles.
3. High Initial Investment
The upfront costs of implementing data analytics can be significant, particularly for small or mid-sized retailers. These expenses may include:
- Purchasing advanced tools and technologies.
- Training staff to work with analytics platforms.
- Upgrading IT infrastructure.
To mitigate costs, retailers should:
- Start Small: Focus on specific, high-impact use cases before scaling analytics efforts.
- Leverage ROI Models: Use analytics to generate early returns and reinvest them in further initiatives.
4. Managing Data Quality
Inaccurate or incomplete data can lead to flawed insights, reducing the effectiveness of analytics. Retailers need to:
- Clean and Validate Data Regularly: Identify and rectify errors, duplicates, or inconsistencies.
- Implement Data Governance Policies: Establish protocols for data accuracy, consistency, and security across the organization.
Robust data quality management ensures the reliability of analytics outcomes.
5. Lack of Skilled Personnel
A major challenge in leveraging data analytics is the shortage of skilled professionals. Key gaps include:
- Data Scientists: Individuals who can process and interpret complex datasets.
- Analytics Translators: Professionals who can convert analytical insights into actionable strategies.
- IT Support: Teams to manage and maintain the analytics infrastructure.
To address this, retailers can:
- Invest in training programs for existing employees.
- Partner with analytics service providers to fill skill gaps.
- Hire talent with experience in retail-specific analytics.
The Future of Retail Analytics in Enhancing Customer Experience
Data analytics will continue to shape the future of retail, offering new opportunities for personalization, efficiency, and customer engagement. Here’s how analytics will evolve in the retail space:
1. Hyper-Personalization with AI and Machine Learning
AI and machine learning will enable real-time, personalized experiences, predicting customer needs and offering tailored recommendations before they’re even expressed.
2. Seamless Omnichannel Experiences
Data analytics will create unified customer profiles across all touchpoints, ensuring personalized and consistent experiences whether online, in-store, or on mobile.
3. AR and VR Integration
Augmented and virtual reality will transform shopping by offering virtual try-ons and immersive storefronts, powered by data analytics to make personalized recommendations.
4. Predictive Analytics for Demand and Stock Optimization
Predictive analytics will enhance inventory management, ensuring optimal stock levels and preparing retailers for seasonal fluctuations, reducing waste and stockouts.
5. Advanced Customer Support Automation
AI-driven customer support will handle more complex queries, while sentiment analysis will help retailers address concerns proactively and improve service quality.
6. Ethical and Transparent Data Practices
Retailers will focus on transparent data usage and give customers more control over their data, building trust and ensuring ethical data collection practices.
7. Real-Time Analytics for Instant Feedback
Real-time feedback systems will allow retailers to monitor customer sentiment and resolve issues immediately, providing a more responsive customer experience.
8. Enhanced Sustainability
Data analytics will promote sustainable products and allow customers to track product origins, fostering ethical purchasing decisions.
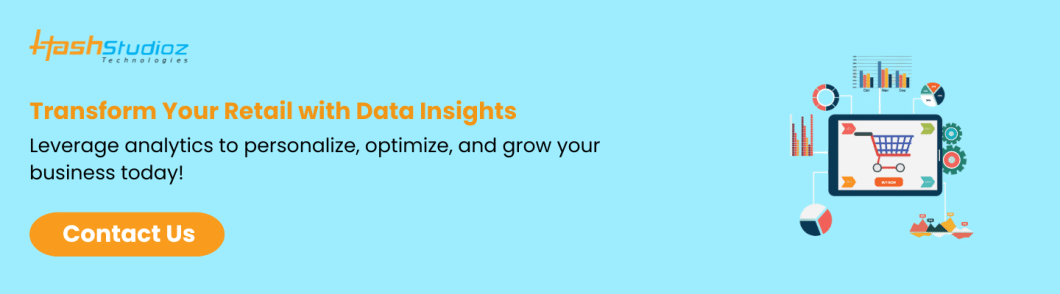
Conclusion
Data analytics is a game-changer for the retail industry. By leveraging data analytics services, retailers can deliver exceptional customer experiences, drive operational efficiency, and achieve long-term growth. However, success depends on strategic implementation, the right tools, and a skilled team.
FAQs
1. What is the role of data analytics in retail?
Data analytics helps retailers understand customer behavior, optimize operations, and make informed decisions to improve customer experience.
2. How can retailers use analytics for personalization?
Retailers can analyze customer data to offer personalized product recommendations, tailored marketing campaigns, and customized shopping experiences.
3. What challenges do retailers face in implementing data analytics?
Key challenges include data security concerns, integration with legacy systems, high initial costs, and managing data quality.
4. What tools are commonly used for retail analytics?
Popular tools include CRM systems, business intelligence platforms, and AI-driven analytics tools.
5. Why is data quality important in retail analytics?
High-quality data ensures accurate insights, leading to better decision-making and improved customer satisfaction.