Every day, over 2.5 quintillion bytes of data are generated, with more than 90% of the world’s data created in the last two years alone. From customer behavior to business transactions, social media interactions to scientific research, data is at the core of many innovations. However, simply having data is not enough to unlock its potential. That’s where Big Data Analytics comes into play.
Big Data Analytics involves examining large and varied data sets to uncover hidden patterns, correlations, trends, and insights that can help organizations make more informed decisions. This article will delve into the tools, techniques, and real-world applications of big data analytics, showing you how it is transforming industries and driving change.
Table of Contents
- What Is Big Data Analytics?
- Tools for Big Data Analytics
- 4 Types of Techniques for Big Data Analytics
- Key Benefits of Big Data Analytics
- Real-World Applications of Big Data Analytics
- Challenges in Big Data Analytics
- The Future of Big Data Analytics
- HashStudioz’s Comprehensive Big Data Analytics Services for Smarter Business Decisions
- 1. Big Data Consulting Services
- 2. Big Data Analytics Solutions
- 3. Custom Data Visualization
- 4. Machine Learning & AI Implementation
- 5. Data Warehousing & Cloud Solutions
- 6. Big Data Architecture Design
- 7. Big Data Security
- 8. Industry-Specific Big Data Applications
- 9. IoT and Sensor Data Analytics
- 10. Big Data Training & Workshops
- Conclusion
What Is Big Data Analytics?
Big Data analytics refers to the process of examining large and complex datasets to uncover hidden patterns, correlations, and trends. These insights help organizations optimize their operations, improve customer experiences, and develop innovative products or services. Big Data is characterized by the three Vs:
- Volume: The amount of data generated is immense.
- Velocity: Data is generated quickly and often in real time.
- Variety: The data comes in many different formats, including structured, unstructured, and semi-structured.
Big data analytics involves processing this massive amount of data to extract meaningful insights and support decision-making.
Tools for Big Data Analytics
The power of Big Data Analytics relies heavily on the tools used to process and analyze the data. Let’s look at some of the most popular tools that businesses and organizations use to harness the potential of big data.
1. Hadoop
One of the most widely used tools for big data analytics is Hadoop. Hadoop is an open-source framework that allows you to process large datasets across distributed computing environments. It is designed to scale from a single server to thousands of machines, each offering local computation and storage. Hadoop provides various components like:
- HDFS (Hadoop Distributed File System)
- MapReduce for parallel data processing
- YARN for resource management
Hadoop is used by major companies to process vast amounts of data in real time.
2. Apache Spark
Apache Spark is another open-source, distributed computing system that is fast, flexible, and highly scalable. Spark is often preferred over Hadoop for real-time data processing due to its in-memory processing capabilities. It supports various programming languages such as Java, Scala, Python, and R, making it accessible to data scientists and analysts.
3. Tableau
When it comes to visualizing big data insights, Tableau is one of the most popular tools. It is a powerful business intelligence tool that allows users to create interactive and shareable dashboards. Tableau can connect to various data sources and allows you to transform complex data into visually appealing charts and graphs.
4. Microsoft Power BI
Power BI is another popular business intelligence tool that enables you to connect, visualize, and analyze big data. It’s integrated with various Microsoft products, making it a great choice for organizations already using Microsoft ecosystems. Power BI’s intuitive interface and drag-and-drop functionality make it easy for users to create customized reports.
5. Apache Flink
Apache Flink is a real-time stream processing platform that is designed for high-throughput, low-latency applications. It allows organizations to process data streams and run analytics in real time, making it a great choice for industries like finance and e-commerce where speed is crucial.
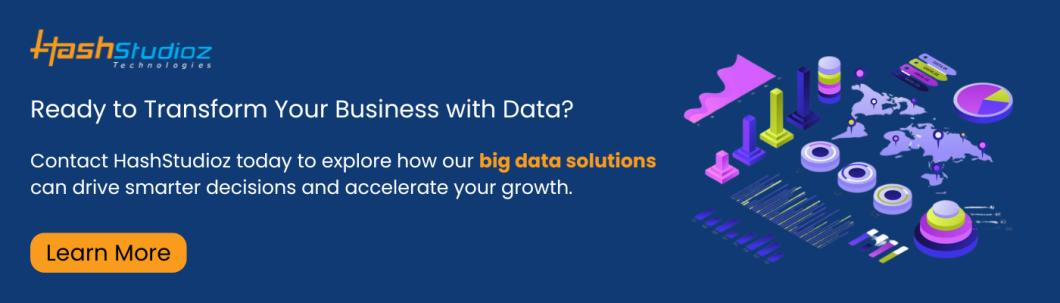
4 Types of Techniques for Big Data Analytics
Once the right tools are in place, it’s time to explore the techniques used to analyze big data. These techniques can provide insights that lead to business optimization, increased revenue, and better decision-making.
1. Descriptive Analytics
Descriptive Analytics focuses on understanding historical data. It answers questions like “What happened?” or “What is the trend?” It provides a summary of past events through various statistical techniques. Examples of descriptive analytics include:
- Data mining
- Dashboards and Reports
- Trend analysis
2. Predictive Analytics
Predictive Analytics uses statistical algorithms, machine learning, and data modeling to predict future outcomes based on historical data. For example, predictive analytics can forecast customer behavior, financial market trends, and equipment failures. It answers the question: “What is likely to happen?”
3. Prescriptive Analytics
Prescriptive Analytics takes predictive analytics a step further by suggesting actions that can be taken to optimize outcomes. It is used to answer the question: “What should we do about it?” It often uses optimization algorithms and simulation models to provide recommendations for the best course of action.
4. Real-Time Analytics
With the rise of the internet of things (IoT) and social media, real-time analytics has become an essential technique. It involves processing and analyzing data as it is generated, allowing businesses to make quick decisions. Real-time analytics is especially valuable in industries like healthcare, finance, and e-commerce.
5. Machine Learning and Artificial Intelligence
Machine learning (ML) and artificial intelligence (AI) are two powerful techniques within the realm of big data analytics. They allow systems to automatically learn and improve from experience without being explicitly programmed. In the context of big data, AI and ML can be used to detect patterns, improve predictive models, and enhance decision-making processes.
Key Benefits of Big Data Analytics
Big data analytics offers several significant advantages to organizations. These benefits can lead to improved business performance, operational efficiencies, and better customer engagement.
1. Improved Decision Making
One of the biggest advantages of big data analytics is that it enables businesses to make data-driven decisions. By analyzing large sets of data, companies can gain insights into market trends, consumer preferences, and potential risks. This leads to more informed and effective decision-making across departments.
2. Increased Operational Efficiency
With big data analytics, businesses can identify inefficiencies in their operations and streamline processes. For example, by analyzing supply chain data, companies can reduce waste, optimize inventory management, and improve logistics. This results in reduced costs and enhanced productivity.
3. Enhanced Customer Experience
Big data allows companies to better understand their customers by analyzing their behaviors and preferences. By using this information, businesses can personalize marketing campaigns, recommend products, and improve customer support. This leads to a better overall customer experience and increased customer loyalty.
4. Competitive Advantage
Organizations that harness the power of big data analytics can gain a competitive edge in their industries. By leveraging data to forecast trends, optimize processes, and deliver personalized services, companies can differentiate themselves from competitors and stay ahead of the market.
5. Innovation and New Business Models
Big data analytics can also spark innovation by revealing new business opportunities. Companies can identify gaps in the market, uncover untapped customer needs, and develop new products or services. Data-driven insights can even lead to entirely new business models that revolutionize industries.
Real-World Applications of Big Data Analytics
Big data analytics is making a huge impact across many industries. Let’s take a look at some real-world examples of how organizations are using big data to drive innovation and solve problems.
1. Healthcare
In the healthcare industry, big data analytics is being used to improve patient outcomes, reduce costs, and streamline operations. By analyzing large datasets of medical records, hospitals can:
- Predict patient health risks
- Optimize treatment plans
- Improve patient care
- Identify trends in disease outbreaks
For instance, IBM Watson Health is using big data analytics to help doctors identify cancer treatments that are most likely to be effective based on patient data.
2. E-Commerce
E-commerce giants like Amazon and Alibaba use big data analytics to enhance customer experience and optimize business operations. By analyzing customer behavior, these companies can:
- Personalize product recommendations
- Predict demand and manage inventory
- Target advertising more effectively
- Improve customer satisfaction through data-driven insights
3. Financial Services
The financial sector is another area where big data analytics is proving invaluable. Banks and financial institutions use big data to:
- Detect fraudulent activity
- Improve risk management strategies
- Optimize trading decisions
- Personalize customer service offerings
For example, American Express uses big data to predict customer spending behavior and offer tailored promotions.
4. Marketing and Advertising
Big data analytics is revolutionizing the way businesses approach marketing. By analyzing consumer behavior across various platforms (social media, websites, etc.), businesses can:
- Create targeted advertising campaigns
- Measure the effectiveness of marketing efforts
- Optimize customer acquisition strategies
For instance, Coca-Cola uses big data to analyze consumer sentiment and make real-time adjustments to marketing strategies.
5. Manufacturing
In manufacturing, big data analytics is used to improve efficiency and reduce costs. By analyzing data from sensors and machines, manufacturers can:
- Predict maintenance needs
- Improve supply chain management
- Optimize production schedules
- Increase quality control
Companies like GE and Siemens are using big data analytics to streamline their operations and create smarter factories.
Challenges in Big Data Analytics
Despite its many advantages, there are several challenges that organizations face when implementing big data analytics.
1. Data Privacy and Security
With the vast amounts of data being collected, data privacy and security are major concerns. Organizations must ensure that they are complying with privacy regulations such as GDPR and CCPA, and that they are taking necessary measures to protect sensitive information.
2. Data Quality
The quality of data is crucial for effective analysis. Poor-quality data can lead to inaccurate insights, which could affect decision-making. Ensuring data accuracy, consistency, and completeness is vital for successful big data analytics.
3. Skill Gaps
The demand for data scientists, analysts, and engineers is growing, but there is a shortage of skilled professionals in the field. Organizations need to invest in training and hiring the right talent to fully leverage big data analytics.
4. Integration of Data Sources
Big data often comes from multiple sources in various formats. Integrating these disparate data sources can be a complex and time-consuming process. Organizations need to invest in tools and strategies to effectively combine data from different systems.
The Future of Big Data Analytics
As the digital landscape continues to evolve, so too will the field of big data analytics. Here are some key trends and developments to expect in the future:
1. Greater Use of AI and Machine Learning
In the coming years, artificial intelligence (AI) and machine learning (ML) will play an even larger role in big data analytics. These technologies will become more sophisticated, enabling businesses to automate complex data analysis processes, improve predictive models, and uncover deeper insights.
2. Real-Time Analytics Advancements
The demand for real-time analytics will only grow. With the expansion of IoT and the increasing number of connected devices, real-time data processing will become more critical than ever. Industries like healthcare and finance will rely heavily on these capabilities to make immediate, data-driven decisions.
3. Enhanced Data Privacy Solutions
As data collection and analysis become more widespread, there will be a growing focus on data privacy and security. Expect to see more advanced solutions designed to protect sensitive information, comply with global regulations, and ensure consumer trust in how data is handled.
4. Democratization of Big Data Tools
The future of big data analytics will involve the democratization of tools. This means that more employees across organizations, even those without technical expertise, will be able to access and use powerful analytics tools. User-friendly platforms will make data analysis more accessible, empowering teams to make decisions based on insights from big data.
5. Integration with Blockchain
Blockchain and big data are two technologies that are beginning to converge. Blockchain can help ensure the security and integrity of data, making it more trustworthy for analysis. This combination will be particularly valuable in sectors like finance, supply chain management, and healthcare.
HashStudioz’s Comprehensive Big Data Analytics Services for Smarter Business Decisions
HashStudioz leverages its expertise in big data analytics to help businesses unlock the full potential of their data. In today’s data-driven world, where vast amounts of information are generated every second, we provide solutions that allow organizations to analyze, interpret, and utilize this data to make smarter decisions. By using advanced analytics techniques and state-of-the-art tools, HashStudioz empowers businesses to gain deeper insights into customer behaviors, operational efficiencies, market trends, and much more.
1. Big Data Consulting Services
- Assessment & Strategy: Help businesses assess their current data infrastructure and create a comprehensive big data strategy tailored to their goals and needs.
- Data Integration: Provide solutions for integrating disparate data sources (e.g., databases, cloud services, IoT devices) into a cohesive big data ecosystem.
2. Big Data Analytics Solutions
- Data Processing & Analysis: Design and implement data pipelines for processing massive datasets and performing advanced analytics.
- Predictive Analytics: Use machine learning and AI to forecast trends and behaviors, allowing businesses to make data-driven predictions.
- Real-Time Analytics: Enable businesses to analyze data in real-time, providing immediate insights for faster decision-making.
3. Custom Data Visualization
- Dashboards & Reporting: Develop interactive dashboards and reporting tools that simplify the interpretation of complex big data insights.
- Advanced Visualizations: Use techniques like heatmaps, graphs, and charts to visually represent data patterns and trends.
4. Machine Learning & AI Implementation
- Model Building: Build machine learning models to analyze large datasets and uncover hidden patterns, correlations, and trends.
- Algorithm Development: Develop custom algorithms to optimize business processes using big data.
5. Data Warehousing & Cloud Solutions
- Cloud Storage & Management: Help businesses migrate their data to the cloud for better scalability, flexibility, and cost efficiency.
- Data Warehousing: Implement data warehousing solutions that consolidate and store data from multiple sources for easy access and analysis.
6. Big Data Architecture Design
- End-to-End Architecture: Design scalable and robust data architectures that can handle large volumes of structured and unstructured data.
- Data Lakes: Build data lakes to store vast amounts of raw data in its native format, ready for processing and analysis.
7. Big Data Security
- Data Encryption & Protection: Implement advanced security protocols to protect sensitive data and ensure privacy compliance.
- Data Governance: Provide data governance services to ensure data integrity, compliance with regulations, and quality control.
8. Industry-Specific Big Data Applications
- Healthcare Analytics: Develop big data solutions for improving patient care, managing healthcare resources, and conducting medical research.
- E-commerce & Retail Analytics: Help retail businesses optimize inventory, personalize customer experiences, and forecast demand using big data insights.
- Financial Analytics: Use big data for fraud detection, risk management, and predictive financial analytics in banking and finance.
9. IoT and Sensor Data Analytics
- IoT Data Integration: Enable businesses to capture and analyze data from connected devices and sensors for operational efficiency and predictive maintenance.
- Smart City Solutions: Provide big data analytics solutions for urban planning, traffic management, and public services in smart cities.
10. Big Data Training & Workshops
- Employee Training: Offer training services to help businesses upskill their teams on big data tools, techniques, and analytics best practices.
- Workshops & Seminars: Conduct workshops on data-driven decision-making, data visualization, and big data technologies.
Conclusion
Big Data Analytics is transforming industries by enabling businesses to make more informed decisions, improve customer experiences, and optimize operations. With the right tools, techniques, and real-world applications, organizations can unlock the true potential of their data and gain a competitive edge. However, challenges such as data privacy, quality, and skill gaps must be addressed to ensure successful implementation.
As big data continues to evolve, it is clear that its impact on business, healthcare, finance, and other sectors will only grow. By harnessing the power of big data analytics, organizations can shape the future of their industries and drive innovation like never before.